Top 5 Challenges in Demand Forecasting and How to Overcome Them
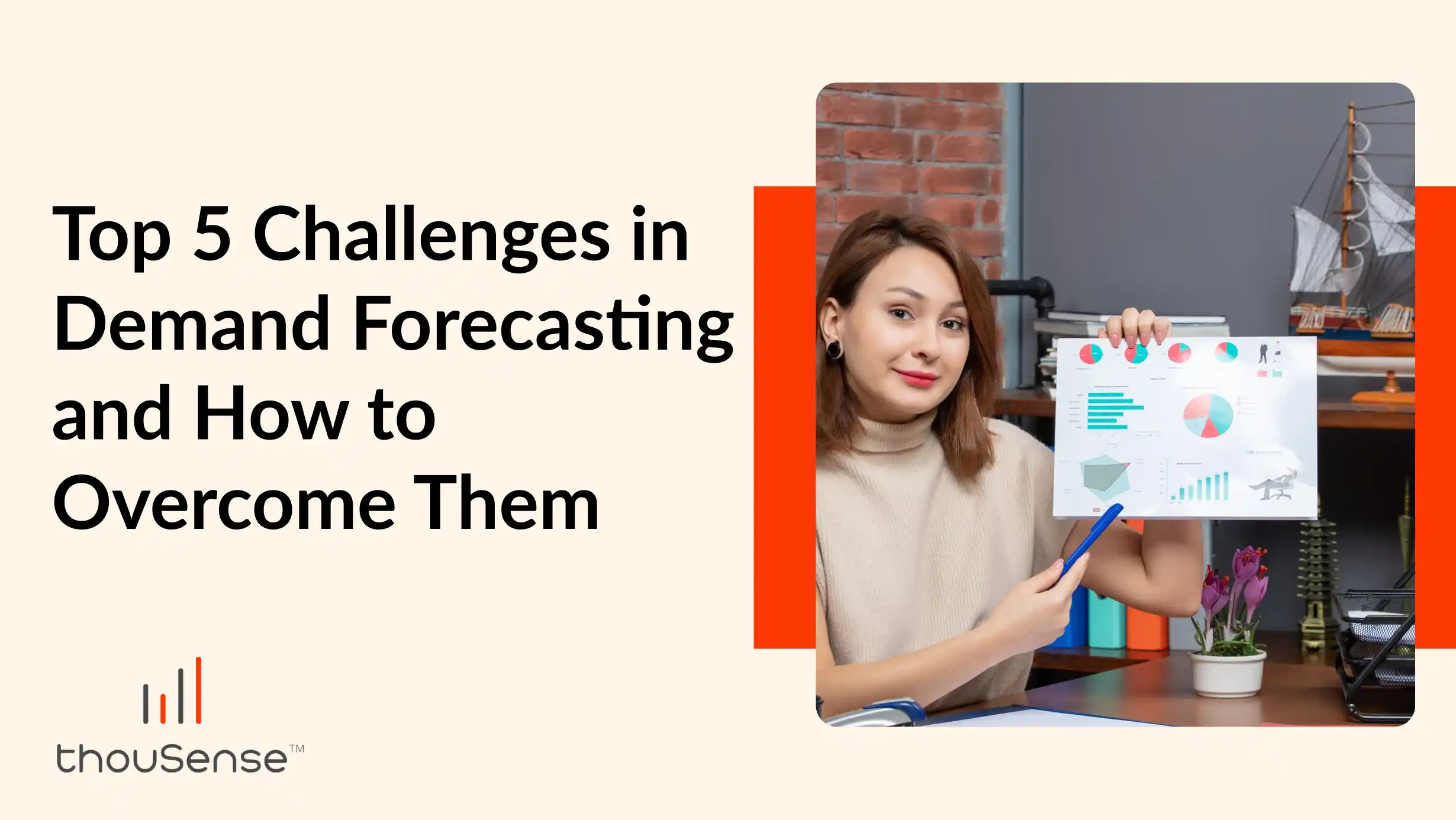
In the ever-changing world of business, being able to correctly predict demand is like having a crystal ball. You could know exactly what your customers want when they need it, and how much they'll need. Surprisingly, demand forecasting is not magic, instead, it is a solution to a difficult process that is full of problems. But knowing about these problems and learning ways to deal with them can make your demand forecasting much more accurate. Let's look at the five biggest problems with demand analysis and forecasting and talk about how to get through these.
5 Challenges in Demand Forecasting
A key part of strategic business planning is demand analysis and forecasting, which is the process of predicting how much of a product or service people will buy. Even though demand forecasting is essential, it can still pose several forecasting challenges to businesses. Why does that happen? Let's look into the challenges of demand forecasting that makes demand prediction difficult.
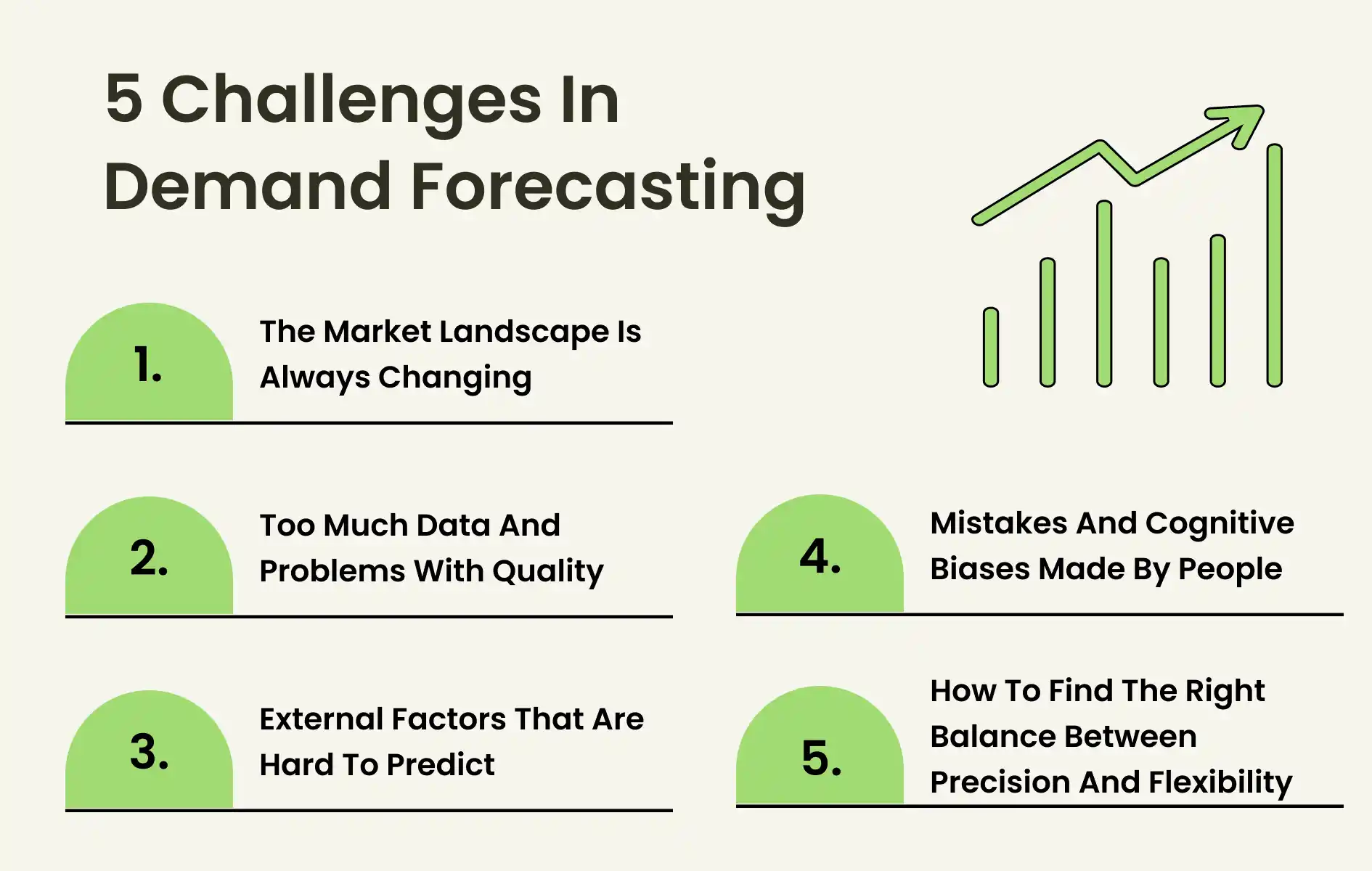
1. The Market Landscape Is Always Changing
One big reason why demand analysis and forecasting is considered so hard is that the market is always changing and moving around.
● Changes in the economy:
The economy is its beast, and its changes can be either regular cycles or sudden events, like the global financial crisis of 2008 or the pandemic that no one saw coming in 2020. These events have a big effect on what people buy and how they act, which makes demand analysis and forecasting very difficult.
● What People Want Keeps on Changing:
Trends, cultural changes, and even content that goes viral on social media can change what people want as quickly as the weather. Ever Changing trends are another factor that gives businesses a strong forecasting challenge since what is cool today might not be trendy tomorrow.
● Improvements in technology:
Because technology changes so quickly, new goods can come out overnight that make old ones useless (think of how streaming services have changed DVD sales). In a world so focused on technology, it's not enough to just have data for demand analysis and forecasting. You also need to know how technology will change in the future.
2. Too Much Data And Problems With Quality
Businesses can get more data now than ever before due to "Big Data." This huge amount of info can be both good and bad.
● More Quantity Than Quality:
Analysts may find it hard to sort through the huge amount of data that is available and find the information that is truly useful for demand analysis and forecasting. This condition of data overloading emerges as yet another demand forecasting challenge as researching becomes impossible.
● Silos Of Data:
Data in organizations is often spread out among different systems and departments. This creates "silos" that make it hard to get a full picture of the data that is needed for accurate demand analysis and forecasting. It is very hard to break down these silos and combine the info they hold.
● Being Accurate And Trustworthy:
Not every piece of info is the same. The data must used for demand forecasting must be correct and reliable. Forecasts that aren't based on good data can be wrong, which can cause overstocking, understocking, and missed chances.
3. External Factors That Are Hard To Predict
Several outside things that are out of a business's control can have a big impact on demand forecasting. Some of these are:
● Instability In Politics:
Elections, trade talks, and war are all examples of political events that can have instant and profound effects on the market, changing consumer confidence and spending habits.
● Unnatural Events:
Things like earthquakes, hurricanes, and pandemics can mess up supply lines and change demand patterns in quite unexpected ways. This makes demand analysis and forecasting very hard.
● Competitive Moves:
When your competitors do things like release a new product or make a big price change, it can have an unpredictable effect on your demand analysis and forecasting.
4. Mistakes And Cognitive Biases Made By People
Demand analysis and forecasting aren't just based on numbers. They also require using your judgment. This makes mistakes and biases more likely to happen.
● Putting Too Much Stock In Past Data:
While looking at past data is important for figuring out trends, making predictions based only on patterns from the past without taking into account how the market is changing now can lead to wrong predictions.
● Confirmation Bias:
People who make decisions may already have ideas about what the forecast should be like, and they may give more weight to data and information that backs up these ideas instead of evidence that goes against them.
● Follow The Leader:
Sometimes, companies make predictions based on what their competitors or the industry as a whole are doing, thinking that those people have more true information. This tendency to follow the crowd can cause everyone to be wrong and the market to work less efficiently.
5. How To Find The Right Balance Between Precision And Flexibility
It's an art to find the right balance between being able to make accurate predictions and being able to change with the times.
● Trying To Get It Right:
Businesses use complex formulas, machine learning models, and AI to try to make the most accurate predictions possible. While trying to be precise, it's important to keep in mind that no prediction can ever be 100% right.
● The Need To Be Able To Change:
It's just as important to be able to respond quickly to changes and make new predictions in real time as it is to try to be accurate. To do this, you need flexible methods, a culture of always learning, and the right technology infrastructure.
Overcoming Demand Forecasting Challenges
Some problems that come up with demand analysis and forecasting are separating data, not having real-time information, using qualitative data, being able to adapt to larger orders, and finding the right mix between service quality and inventory levels.
The fact that businesses separate their data into different areas makes it harder to properly predict what the market will want. When different teams work on their own, the data they collect is only useful for their areas. This makes it hard to get a full picture of the market demand analysis, contributing itself as demand forecasting challenges.
This split-up way of managing data often causes strategies that don't work with each other in different parts of the business. For example, marketing efforts that don't match up with stock levels. This difference can make it much harder to get accurate demand estimations.
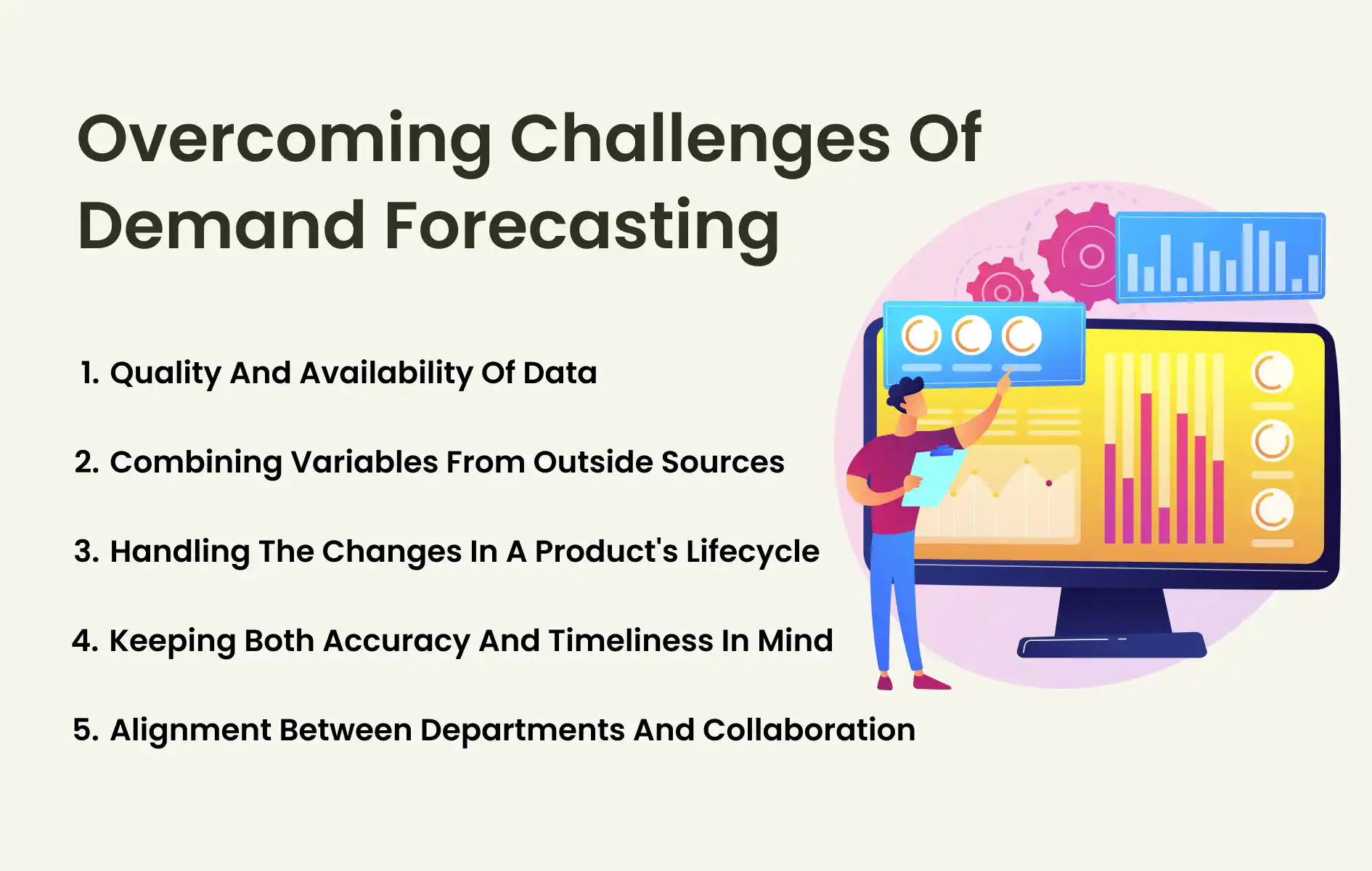
1. Quality And Availability Of Data
Good data is the basis of any demand-predicting model that works well. But businesses often have to deal with data that is missing, wrong, or out of date, which can make predictions that aren't correct.
Solutions:
● Data cleanup: Data cleanup means checking your data sources often to find mistakes and fix them.
● Use tech to your advantage: Using advanced data management tools and demand forecasting tools can make data cleanup and integration automatic, making sure that you always have a steady flow of correct data.
● Add more data sources: Don't just look at past sales numbers. To improve your forecasting, use outside factors like market trends, economic indicators, and social media opinion.
2. Combining Variables From Outside Sources
Demand estimation can be greatly affected by things outside of the company, like the economy, what competitors do, and changes in what customers want. A lot of the time, traditional forecasting methods can't take these changing factors into account, which makes their predictions wrong.
Solutions:
● Advanced Analytics: Use advanced tools like thouSense to look at and predict how outside factors will affect demand analysis and forecasting.
● Planning For Scenarios: Create and update scenarios regularly that take into account different outside factors. This will let you quickly change your predictions as things change.
● Collaboration and Insight: Build relationships with experts in your field and use customer feedback routes to learn about outside factors that could affect your analysis and demand forecasting.
3. Handling The Changes In A Product's Lifecycle
As innovation speeds up, product life cycles are getting shorter. Because of this, it's hard to predict demand, especially for new goods that don't have any historical data.
Solutions:
● Use the same product analysis: For new product demand models, look at data from similar goods or products that have already come out.
● Pre-launch Testing: To get an idea of what people will want first, do a market study and pre-launch tests.
● Report and Evaluation: After the product is released, it should be closely watched for sales and predictions should be changed based on real-time data. Being flexible is important for dealing with sudden changes in a product's existence.
4. Keeping Both Accuracy And Timeliness In Mind
It's not always easy to balance the need for accurate predictions with the need to make them on time. Businesses need to find a balance between doing thorough, accurate demand forecasting, and giving quick and useful insights.
Solutions:
● Forecasts that change over time: Instead of static forecasts that are made once a year, use rolling forecasts that are changed regularly. This lets changes be made more quickly based on the most recent information.
● Step-by-Step Method: Make short-term predictions that are very specific, and long-term predictions that are more general and not as specific. This plan lets you be precise where it matters most while still giving you options for making long-term plans.
● Accept Uncertainty: Use both range forecasting and probabilistic forecasting to give a range of possible results, while keeping in mind that you can't be completely accurate.
5. Alignment Between Departments And Collaboration
Demand forecasting shouldn't happen in a vacuum. When departments like marketing, sales, finance, and operations aren't working together properly, it can cause problems with predictions and, in the end, the business plan.
Solutions:
● Processes for Integrated Planning: Set up a way for all the important people involved in demand planning to work together. This shared method makes sure that all parts of the business are taken into account in forecasts.
● Using technology together: Use collaborative demand forecasting tools and platforms that let departments share forecasting data and ideas in real time.
● Review and Change: Hold cross-functional talks regularly to go over forecasts, share new information, and make changes to plans as needed. This keeps everything in line and lets you act quickly when new information comes in.
Addressing Demand Forecasting Challenges
To tackle the obstacles of different forecasting challenges, companies might employ conventional demand prediction tools such as time series analysis, moving averages, and exponential smoothing. These instruments, by analyzing past data, forecast future demand and provide insightful information for making strategic decisions.
Yet, to achieve more precise and efficient demand forecasting, companies can switch to sophisticated AI-powered solutions like thouSense. thouSense harnesses the power of advanced artificial intelligence and machine learning algorithms to swiftly and accurately sift through massive datasets.
The Edge of AI in Predictive Demand Forecasting
At the core of AI-enabled forecasting technologies is their unmatched proficiency in sifting and analyzing huge data volumes at astonishing speeds, an endeavor that human analysts find beyond their reach. However, the prowess of AI in prediction goes much deeper than simple data analysis. Here, we delve into these groundbreaking elements more thoroughly.
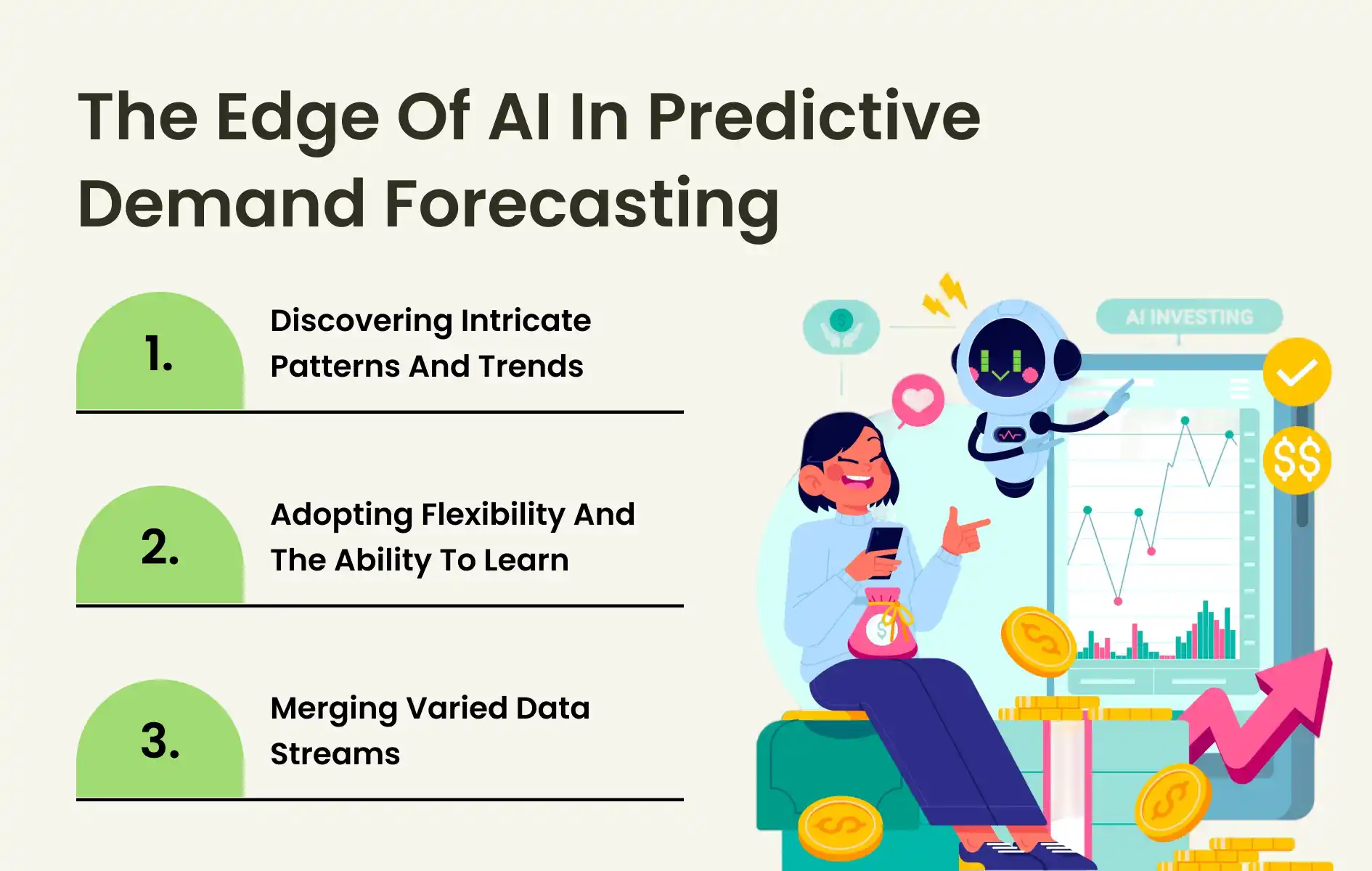
● Discovering Intricate Patterns and Trends
AI/ML-based algorithms are particularly skilled at detecting non-linear connections and subtle intricacies within extensive data sets. This skill encompasses grasping the complex interactions among various demand-influencing factors, such as seasonal fluctuations, economic signals, and the mood on social media platforms. Utilizing this aptitude, AI prediction tools can furnish a more detailed and precise forecast of future demand.
● Adopting Flexibility and the Ability to Learn
A notable attribute of AI forecasting solutions is their capacity to learn and adjust dynamically over time. With the influx of new information, these systems fine-tune their algorithms to enhance precision, a feat that traditional models struggle to achieve. This attribute of continuous improvement ensures that the forecasting system remains up-to-date with changing market dynamics.
● Merging Varied Data Streams
In the contemporary digital realm, valuable data can originate from numerous sources, ranging from conventional sales figures to web traffic and interactions on social media. AI-powered mechanisms excel at amalgamating these varied data inputs, offering a comprehensive insight into the demand scenario. This broad-based approach enables companies to consider external elements, like marketing initiatives or competitive moves, in their demand projections.
Advantages of Using thouSense for Demand Forecasting
With the help of artificial intelligence, thouSense AI's innovative Demand Sensing technology is changing the way that demand forecasting and forecasts are done.
This method makes it possible to accurately predict what customers will want in the short term, so businesses can respond quickly to changes in the market.
By combining past data with outside factors like weather and seasonal trends, thouSense gives users a more accurate picture of what customers will want in the future.
This advanced method goes beyond basic demand forecasting tools because it not only figures out what people want, but also gives them useful ways to meet those needs quickly.
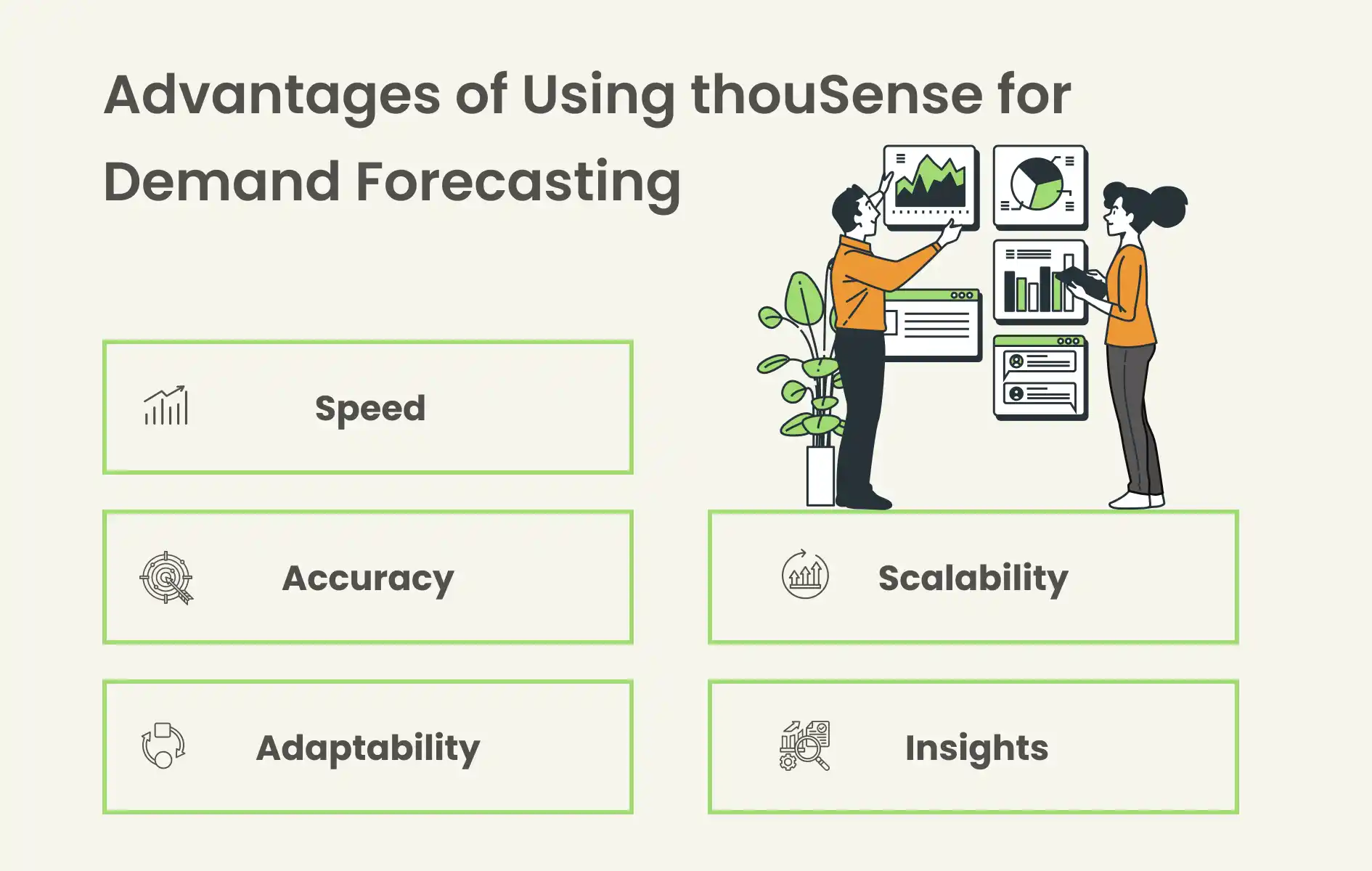
● Speed: thouSense swiftly analyses data, facilitating instantaneous forecasting and allowing businesses to quickly adjust to fluctuating market trends.
● Accuracy: By using sophisticated algorithms, thouSense offers forecasts with high accuracy, reducing mistakes and enhancing the quality of decisions.
● Adaptability: thouSense adjusts to changing demand patterns and market situations, guaranteeing consistent forecasting accuracy over time.
● Scalability: thouSense effortlessly scales, regardless of whether it's forecasting for a single item or a vast array of products, meeting the requirements of companies of every size.
● Insights: thouSense goes beyond mere forecasting by offering actionable recommendations and insights to improve inventory management, production scheduling, and the efficiency of supply chain operations.
Conclusion
Demand forecasting is difficult because businesses have to deal with poor data quality, outside factors that can change things, unstable product lifecycles, accuracy vs. timeliness problems, and the need for everyone to work together. Businesses can greatly improve the accuracy of their forecasts, though, by recognizing these problems and putting in place strategic answers.
FAQs
1. How do mistakes made by people and cognitive flaws affect demand forecasting?
When people make predictions about demand, they might make mistakes like relying too much on past facts, confirmation bias, and following the crowd. These biases can cause predictions to be wrong and markets to work less efficiently.
2. How can businesses get around problems with demand forecasting?
To get around the problems that come with predicting demand, businesses can use advanced analytics, combine different data sources, adapt to changes in the market, and encourage a mindset of making decisions based on data.
3. What part does technology play in making demand forecasts more accurate?
AI/ML- based tools can improve the accuracy of demand forecasting by quickly going through huge amounts of data, finding trends, and making more accurate predictions about how people will behave in the future.
4. What are the biggest challenges in forecasting the revenues?
There are several forecasting challenges that can come into light while forecasting revenues. The major demand forecasting challenges involved with revenue generation are lack of predictive data and low accuracy of past data. Addressing revenue forecasting problems with solutions in an appropriate manner is necessary.
5. What is the main challenge of forecasting demand?
Demand forecasting challenges involve aspects like lack of qualitative data integrations, inaccuracy due to limited historical data and failure to understand seasonality. Companies should opt for the support of better technologies to cater the forecasting challenges.