From Reactive to Proactive: Leveraging AI/ML in Demand Sensing
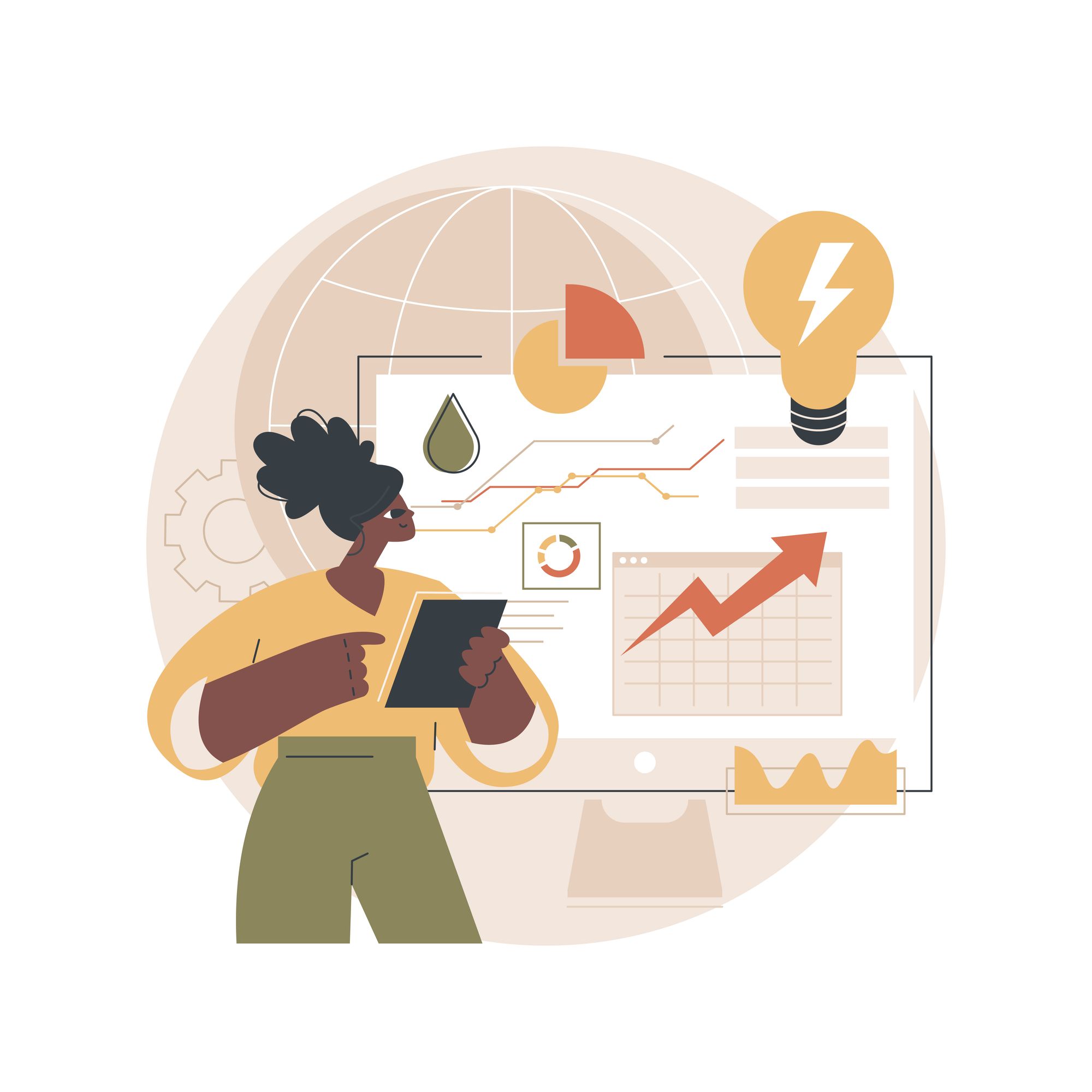
The retail industry has gone through some major transformations recently, thanks to advancements in technology and evolving consumer behaviors. In this ever-changing landscape, demand forecasting has become a critical aspect of retail operations.
So, why is demand forecasting so important in retail? Well, accurate demand forecasting helps retailers optimize inventory levels, reduce stockouts and excess inventory, and ultimately enhance customer satisfaction. But here's the thing: traditional demand forecasting methods struggle to keep up with the complexity and dynamism of today's retail environment. That's where artificial intelligence (AI) steps in to save the day.
Now, let's dive into the fascinating world of AI and machine learning (ML) and how they can take retailers from reactive to proactive demand planning. By taking the power of AI/ML, retailers can stay ahead of the competition and make more informed decisions. Exciting, right?
The Importance of Demand Forecasting in Retail
But wait, let's take a step back and ask ourselves a question: What makes demand forecasting so crucial in the retail industry? Well, the answer lies in the fact that demand forecasting helps retailers optimize cash flow, minimize lost sales, and strike a balance between excess inventory and stockouts.
Moreover, it allows retailers to respond promptly to consumer trends, giving them a competitive advantage and creating an enhanced shopping experience.
Reactive to Proactive Demand Planning
Now, in today's fast-paced retail landscape, relying solely on historical data for demand planning is no longer sufficient. Retailers need to adopt proactive demand planning strategies that allow them to anticipate market fluctuations, respond to evolving consumer preferences, and make data-driven decisions.
This is where AI-driven demand sensing comes into play, empowering retailers to proactively optimize their operations and achieve long-term success.
Traditional Demand Forecasting Methods and their Challenges
Let's examine the problems with conventional demand forecasting techniques in more detail. Although these techniques have been useful to the retail sector for many years, they are constrained by the current retail landscape.
Significant obstacles are posed by elements including quickly shifting consumer tastes, expanding e-commerce, and intricate supplier networks. Forecasts made using conventional approaches are frequently wrong since they don't take into account outside variables like competition pricing and local occurrences.
Additionally, the sheer volume of data generated from multiple store locations, distribution centers, and sales channels overwhelms traditional methods, making it difficult to maintain forecast accuracy.
Advantages of AI/ML in Demand Sensing
Alright, let's turn our attention to the advantages of AI/ML in demand sensing. AI-driven demand sensing brings several benefits to retailers. Machine learning algorithms can analyze large datasets and identify patterns and correlations that are difficult for humans to discern.
This means AI systems continuously adapt to new information, refining predictions and enabling retailers to anticipate future revenue, tailor marketing efforts, and streamline inventory management.
By leveraging AI/ML, retailers can optimize supply chain planning and enhance overall sustainability by reducing waste and operating more efficiently.
AI Techniques in Retail Demand Sensing
Now, let's talk about some cool AI techniques used in retail demand sensing. Machine learning, statistical analysis, and the integration of external data sources are just a few of the methodologies used to create comprehensive, data-driven forecasting systems.
These techniques enable retailers to improve future demand prediction, support various planning processes such as inventory management and promotion planning, and enhance the overall customer experience. Isn't it fascinating how AI can transform the way retailers operate?
Implementing AI/ML in Demand Sensing
Implementing AI/ML in demand sensing requires a comprehensive approach. Retailers need to integrate advanced analytics capabilities, data infrastructure, and domain expertise to build effective AI-driven demand sensing solutions.
Here are key steps to successfully implement AI/ML in demand sensing:
● Data Collection and Preparation:
The first step is to gather and organize relevant data from various sources such as sales transactions, inventory levels, market trends, social media, and external factors like weather and events.
This data needs to be cleansed, standardized, and prepared for analysis. Advanced data management techniques, including data integration, transformation, and quality assurance, are essential to ensure accurate and reliable forecasts.
● AI Model Development:
Once the data is prepared, retailers can leverage AI techniques such as machine learning, time series analysis, and predictive modeling to develop demand forecasting models.
These models can be trained using historical data to identify patterns, seasonality, trends, and other factors that impact demand. The models should be flexible enough to adapt to changing market dynamics and incorporate real-time data for continuous learning and improvement.
● Integration of External Data:
To enhance the accuracy of demand forecasting, retailers can integrate external data sources such as competitor pricing, economic indicators, social media sentiment, and industry reports.
By incorporating this information into the forecasting models, retailers gain a holistic view of market conditions and can adjust their strategies accordingly.
● Collaborative Planning:
Successful demand sensing requires collaboration between different departments within the organization, including sales, marketing, supply chain, and finance.
By involving stakeholders from across the organization, retailers can gather diverse insights, align strategies, and ensure that demand forecasts are integrated into decision-making processes effectively.
● Real-Time Monitoring and Adjustment:
AI/ML-based demand sensing solutions enable real-time monitoring of key metrics and demand indicators. Retailers should regularly evaluate the accuracy of their forecasts and adjust based on new information or changing market conditions.
This iterative process allows for continuous improvement and ensures that forecasts remain relevant and reliable.
● Performance Measurement:
It is crucial to measure the performance of AI-driven demand sensing solutions against predefined metrics such as forecast accuracy, inventory turnover, stockouts, and revenue growth.
By regularly assessing the performance, retailers can identify areas of improvement, fine-tune their models, and optimize their forecasting capabilities.
Challenges and Considerations
Implementing AI/ML in demand sensing also comes with its challenges and considerations. Some key points to keep in mind include:
● Talent and Expertise:
Building and deploying AI/ML models requires skilled data scientists, analysts, and domain experts.
Retailers may need to invest in hiring or upskilling their workforce to effectively leverage AI/ML in demand sensing. Collaboration with external partners or consultants can also be beneficial in accessing specialized expertise.
● Ethical Considerations:
As AI-driven technologies become more prevalent in demand sensing, retailers should consider ethical implications such as data privacy, bias, and transparency.
It is important to establish ethical guidelines and practices to ensure responsible use of AI/ML and maintain customer trust.
● Scalability and Integration:
AI/ML solutions should be scalable to handle large volumes of data and support the growth of the retail business. Integration with existing systems and processes, such as enterprise resource planning (ERP) and supply chain management (SCM), is crucial for seamless operations and data flow.
Conclusion
AI/ML has the potential to revolutionize demand sensing in the retail industry. By leveraging advanced analytics, machine learning, and real-time data, retailers can improve demand forecasting accuracy, optimize inventory management, and enhance customer satisfaction.
However, successful implementation requires a strategic approach, data-driven decision-making, cross-functional collaboration, and a commitment to continuous improvement. With the right tools and expertise, retailers can gain a competitive edge in a dynamic and rapidly evolving market.