Mastering demand forecasting: Tips and tricks for business owners
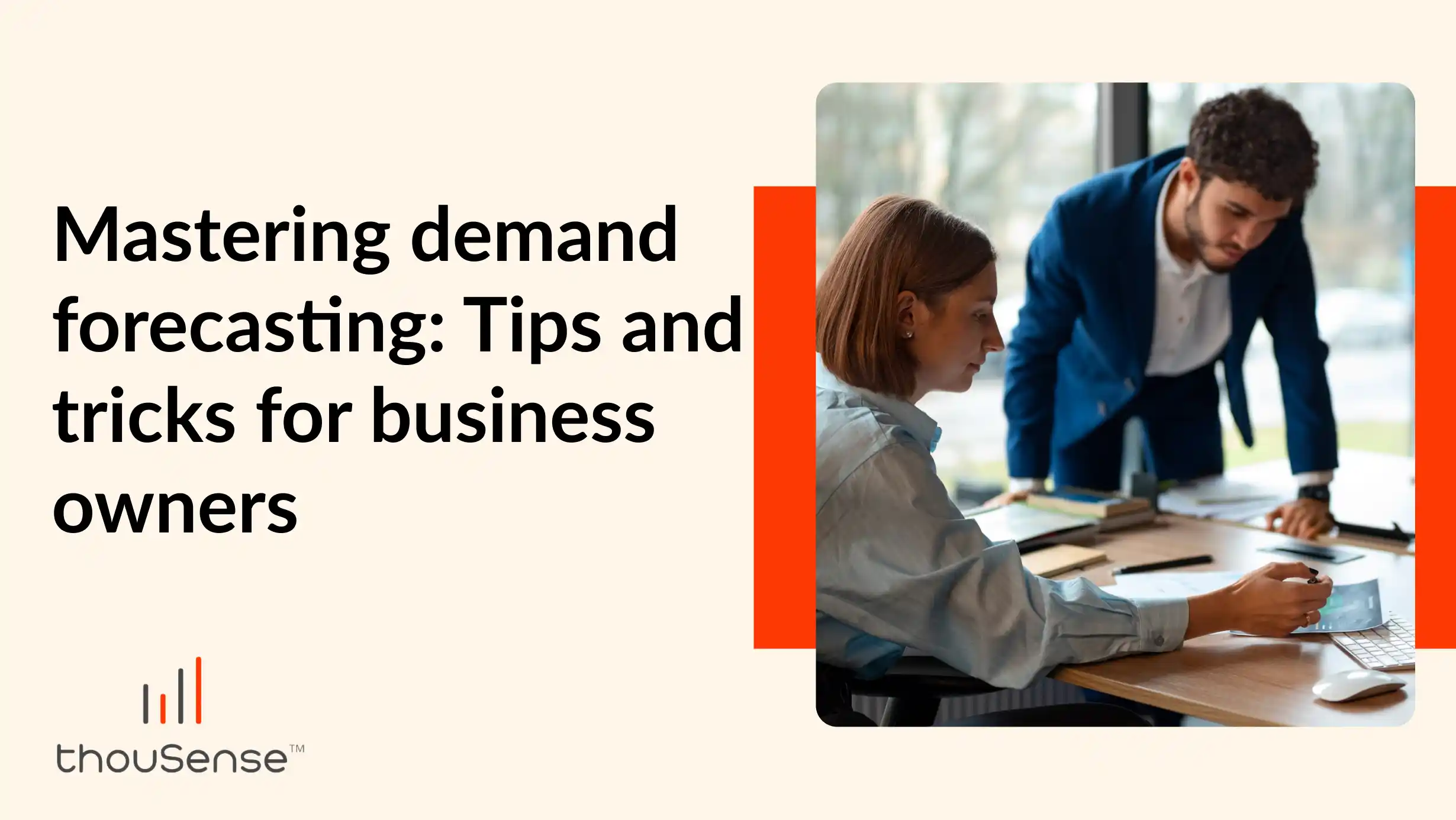
For the majority of businesses, from retailers to distributors to vendors, understanding client demand is essential to making successful decisions. But how can you tell what your potential customers want and need?
Business owners can make educated predictions about upcoming patterns in consumer behavior by utilizing demand forecasting strategies. By doing this, supply choices are based on reliable information rather than wild guesses.
What is the forecasting of demand?
The technique of predicting future demand for goods and services is known as demand forecasting. Making more precise and realistic predictions about purchase trends is feasible by examining past sales data or external influences.
Demand forecasting comes in a variety of forms, and the method you choose will largely rely on the objectives and resources of your company. Using data from previous sales, passive demand forecasting makes future predictions. On the other side, active demand forecasting enables enterprises to take into current market analysis and outside factors, such as the overall economy.
Foreseeing the long term is crucial to a company's success. Capital expenditures, business functions, allocation of resources, and marketing techniques are essential in achieving sustainable corporate growth.
Forecasting Demand's Restrictions
These are the demand forecasting's limitations:
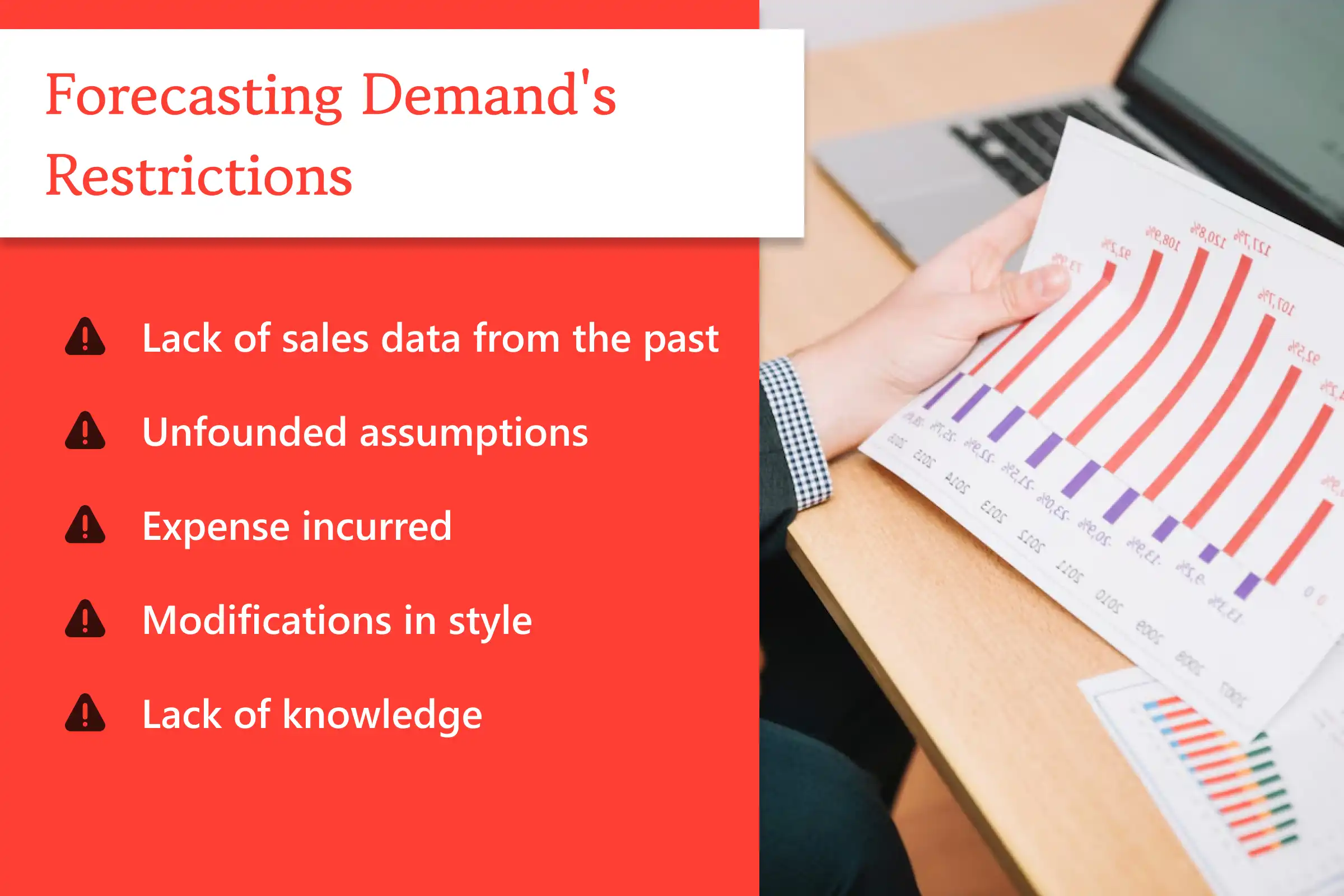
1. Lack of sales data from the past
2. Unfounded assumptions
3. Expense incurred
4. Modifications in style
5. Lack of knowledge
Lack of sales data from the past
An organization might not always have access to past sales data. For instance, there are no previous sales figures available for new commodities. In these situations, new data must be gathered for market research, which can be time-consuming and difficult for an organization.
Unfounded assumptions
Demand forecasting is dependent on several assumptions, some of which may not be true given the state of the market. In this situation, depending on these assumptions could result in inaccurate future projections.
Expense incurred
Demand forecasting comes with a variety of expenses for an organization, including implementation, labor, and administrative expenditures. Depending on the intricacy of the forecast method chosen and the resources used, these costs could be very significant. Demand forecasting becomes challenging for new small businesses and startups due to restricted resources.
Modifications in style
With changes in fashion, consumer preferences and tastes continue to evolve. As demand forecasting is typically dependent on previous trend research, this restricts its application.
Lack of knowledge
Demand forecasting requires forecasters to have the necessary expertise, knowledge, and abilities. Demand forecasting becomes difficult for an organization when there are no qualified specialists available.
Artificial intelligence and machine learning help to overcome predicting limitations
AI is quickly gaining ground in the supply chain, especially for demand forecasting. AI minimizes unpredictable inventory purchases, excess stock (and the resulting markdowns), out-of-stocks, profit erosion, and the optimization of new waste when utilized effectively.
AI-based forecasting systems use machine learning, which is founded on the idea that when we give machines data, they can understand on their own. Machine-learning algorithms are automatic, allowing them to examine all data, not just a subset of it, at scale.
In conventional demand forecasts, data is sent to a computer, which then analyses it and produces a result by applying the data to a static, predetermined set of criteria. With machine learning, however, the computer becomes adaptive, responding dynamically to data changes and altering the projections to reflect them. Businesses can respond to customer demand more swiftly over time thanks to the huge increase in accuracy that results from this.
Data is made competitively advantageous via AI
Machine learning-based AI prediction will increasingly be the standard for projecting retail demand in the future. Instead, the system serves as an independent data scientist, providing additional levels of data, alerts, and conclusions for your data.
Additionally, AI isn't just for the biggest retailers. Retailers of all sizes can now use AI to their advantage and free up their supply chain supervisors to focus on more strategic tasks thanks to the development of innovative Software as a Service (SaaS) solutions.
AI assists retailers with more accurate, automated pull-based replenishment as well as push-based replenishment that is more profitable due to enhanced forecasts. Supply chain managers can adapt to rapid changes in demand because of the algorithms' ongoing learning and ability to provide real-time views as well as weekly and intraday projections.
In the end, AI-based demand forecasting drives total shopper happiness by fostering happy and devoted customers.
Practices in Demand Forecasting
There are numerous ways to forecast demand within the realm of both qualitative and quantitative forecasting. The best option will depend on your company's needs, therefore evaluating each approach is the first step.
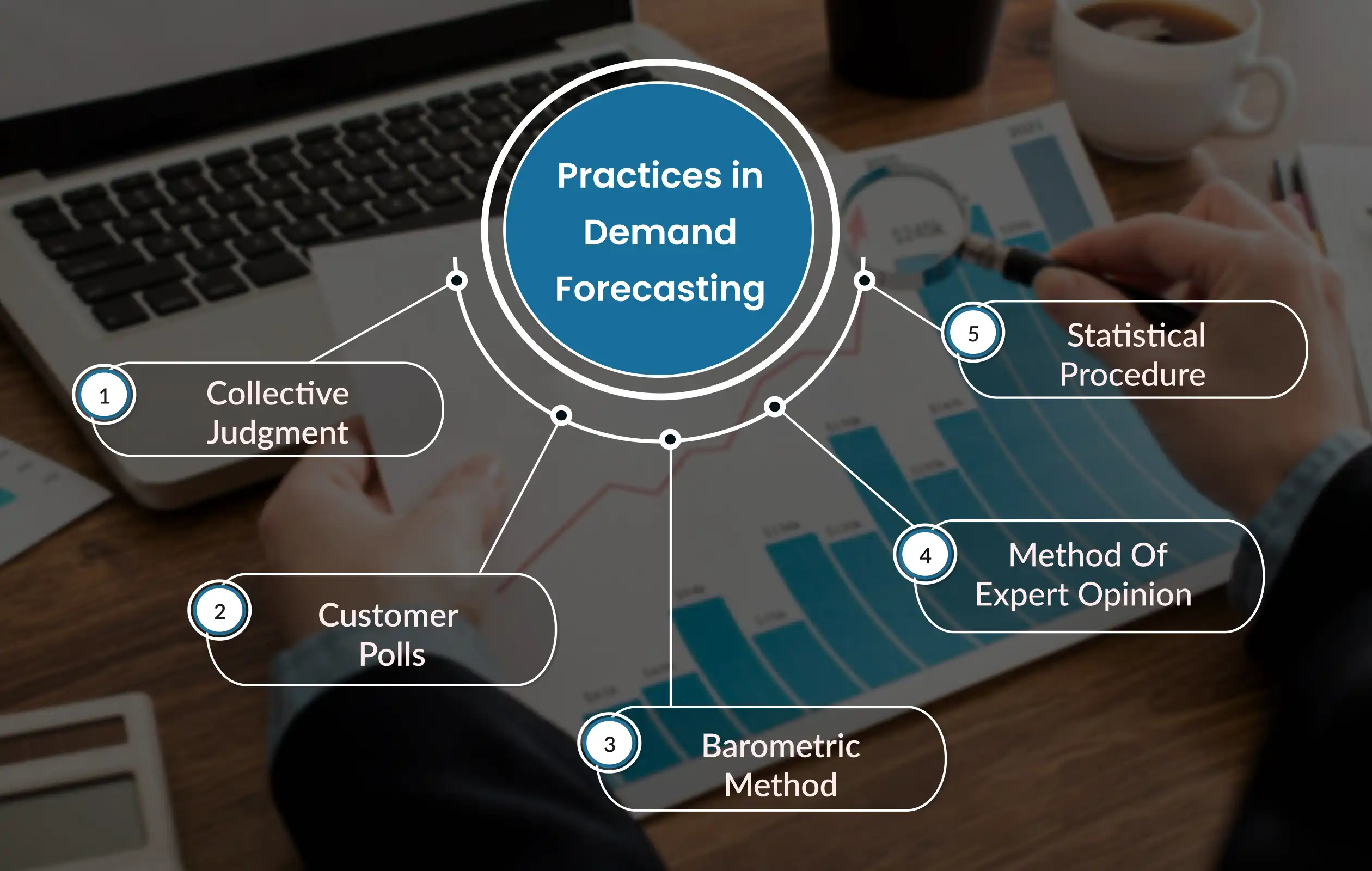
1. Collective judgment
Utilizing the knowledge and skills of a company's sales personnel, the aggregate opinion approach to data forecasting gathers information about client demand. Sales team members provide reports on the sales success of certain items in their assigned regions, enabling you to draw wider conclusions about overall demand from more specific data.
Sales executives can typically acquire and evaluate this data using built-in features of inventory management solutions to optimize their seasonal inventories. The costs of a product, associated marketing initiatives, job prospects, customer economic information, and nearby competitors are all taken into consideration.
2. Customer polls
Customer surveys can offer important details about the requirements, wants, and expectations of customers. To better understand the consumer base, this type of market study also looks at the economic and demographic characteristics of certain customers.
3. Barometric Method
Economic data are used in the barometer approach to forecasting trends.
3.1 Benchmarking is a process that may foretell future events as a leading indicator.
3.2 Lagging indicator: A performance indicator for the past that examines the effects of the past.
3.3 A measure of current affairs that are occurring in real-time or over a brief period is a coincidental indicator.
We can take advantage of these signs in a variety of ways. A sudden increase in sales could be a sign that the economy is improving. Businesses can use trailing indicators like retention and growth to provide additional context. The surge may be an exception or the start of a trend. For better inventory and supply chain management, all of these variables can be utilized to assess past, present, and future business activities.
4. Method of Expert Opinion
To decide on future action, you can ask external contractors for qualified assistance. Market experts employ a variety of techniques, including the Delphi method, which consists of a series of surveys intended to collect the data required to make forecasts.
After engaging in brainstorming with the pertinent professionals, conclusions can be drawn that will help your business plan for the upcoming days, weeks, or even years. This approach may be both time and money-efficient, enabling businesses to easily deploy it.
5. Statistical Procedure
Statistical techniques are thorough, trustworthy, and frequently economical. Several types of statistical techniques are as follows:
Regression Analysis: This technique enables a business to find and examine the connections between various variables.
Trend Analysis: To determine performance history across time, analyze trends, and forecast potential future trends, this strategy uses larger data sets of prior sales.
Sales, registrations, and email signups are just a few examples of the variables used in regression analysis. A business can better allocate funds to the right areas and increase sales by taking a comprehensive look at how each is impacted by the other. A useful technique for better inventory management is trend prediction, which forecasts demand for certain seasons of the year.
Conclusion
Collecting your data is only half the battle, of course. You must also act on that data by implementing it into the business model, as McKinsey Digital emphasizes. You must encourage a data-driven environment within your business. If the forecast is new for you, have a staff meeting to discuss the best way to apply the information you've learned from your study.
When implementing demand forecasting for the first time, investing in staff training or engaging a consultant can be beneficial, however, occasionally it only requires careful planning among your internal team.
You may successfully enhance revenues and reduce wasteful expenditures by enhancing demand forecasting and supply chain optimization. Choose the technologies that will assist you in precisely calculating demand after taking a close look at your organization and determining which techniques suit your sector the best. By putting this strategy in place, you can increase the flow of your inventory and get the resources you need to advance your e-commerce firm.
Thousense is here to help you with solutions to your end-to-end demand forecasting problems. To learn more about the benefits and solutions we can provide you, visit us at https://lite.thousense.ai/