4 Mistakes that Retail Companies Make While Forecasting Demand
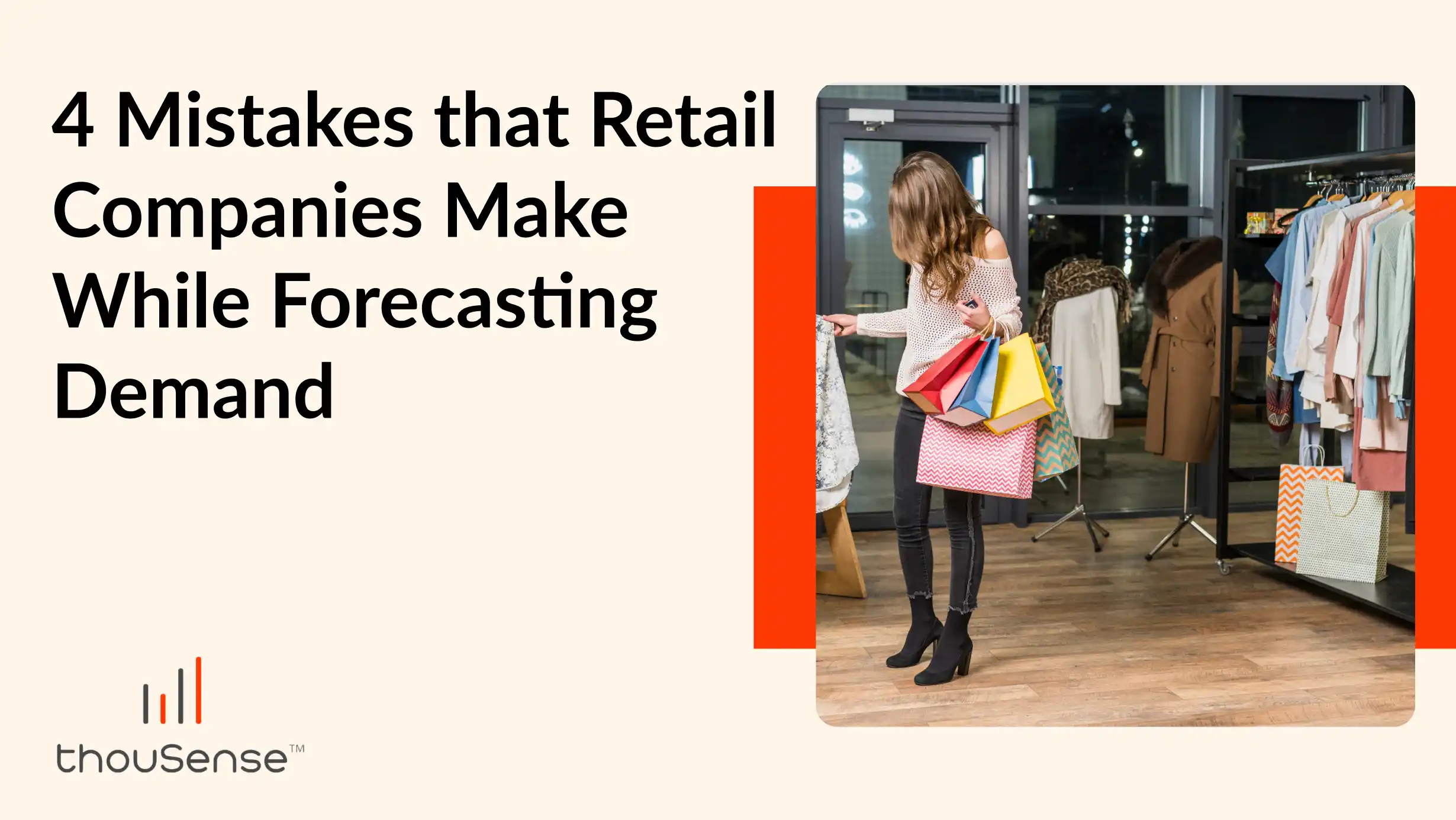
Demand forecasting in the retail industry
The retail industry is an ever-green industry catering to a wide range of product categories. From grocery stores to life stores all retailers rely on forecasting products which helps them in gaining profit. Today, it is incredibly challenging for businesses to generate profitable and sustainable growth due to unpredictable buyer behavior, a complicated supply chain network, changing market conditions, and intense competition. Predicting the demand and providing the right supply of products is crucial.
There are many ways with which one can predict demand which include predictions, analyzing the previous year's performance, excel or spreadsheet-based formulae calculations and the list goes on. Since there are so many options available in today's market, the retail industry needs to make sure that customer experience is never compromised due to a lack of Product availability. For Retail businesses, Accurate forecasting is always intended for better product availability, reduced safety stock, less wastage, higher margins, and lower operating costs. For them, product availability is important to be able to fulfill the customer demand on time and reduce the customer churn rate. While on the one hand accurate forecasting enables retail businesses to better predict, plan, and perform on the other hand inaccurate forecasting can lead them to a great loss.
Having said that, do the best retail market leaders use these traditional ways? Not at all! They use smart predictive tools such as Artificial Intelligence and machine learning-based forecast tools. For instance, retail planogram planning and execution based upon the supply chain demand, seasonal trends, and other external factors is tedious. But with an intelligent tool like machine learning-based demand forecasting, anything and everything is possible.
The most prevalent mistakes committed by retail organizations when estimating demand are listed below:
Inability to understand the importance of Sales forecast VS Demand forecast
While forecasting, the retail sector frequently makes errors because it confuses the terms demand forecast and sales forecast, though there is a very fine line between these two.
Demand forecasting assists you in capturing true unconstrained demand that considers your customers' unfulfilled demand. The sales forecast, on the other hand, is primarily based on your past POS (Point of Sales) data and does not account for sales that were lost because a product was out of stock when a consumer was placing an order.
For instance, imagine that you were consistently selling a certain product in pretty good numbers. However, due to a production problem, a supply problem, or the possibility that your product was understocked, they went out of stock for some time. As a result, the demand that was recorded during that time was not your actual demand for the product; it might have been different if your product had always been available, and this will lead you to calculate the depressed demand of that out-of-stock product for future calculation. Incorporating product availability as one of the KPIs in your forecasting model has become the most important component of any demand forecasting model. Calculating the lost sale due to the unavailability of the product and incorporating these scenarios into your forecasting model will save you from ending up with understocking of your high-performing products and eventually losing your potential customers and company profit.
Inability to incorporate effective demand drivers into the forecasting model
Traditionally retailers used to look only for historical data to predict future demand but nowadays It is not enough as demand planning is entirely different due to constantly changing demand drivers. Business leaders must consider numerous internal and external variables or factors to produce projections that are as accurate as feasible.
Demand is directly impacted by internal factors such as promotions, price changes, assortment modifications, new product introductions, etc, and external factors such as holidays, regional festivals, competitors’ strategies, government rules, and weather conditions (for example short-term weather forecasts which may affect replenishment of your fresh food category items) even though there are millions of data available, many businesses are unsure of where to start looking to identify these factors or drivers influencing business success. Eventually, they end up using their old Forecasting model which neither improves the accuracy nor gives them the desired business result
Forecasting conglomeration
In traditional forecasting, demand for the products is created by analyzing the past sales data and with native intelligence. It is impossible to use it in the current scenario. Hence, the use of forecasting tools emerged. Despite that, it is mandatory to predict demand based on various categories for a particular timing frequency. For instance, just predicting a monthly demand or a yearly demand for a product and sticking with it for the long run is not advisable. Demand forecasting should be used by a team of departments, not just one. For example, the business analyst team should analyze the current market trend, the marketing team should increase the demand for a product based on trending campaigns, and the logistics team must determine how many SKUs require products at what time. As the trends are changing frequently, it is wise to demand a plan every week if needed. To understand the high level of current trends and market data, a business should need an artificial intelligence-based demand forecasting tool.
Other Trivial Mistakes in Demand Forecasting
Minor errors include failing to correctly analyze past sales data. Every business runs uniquely. Choosing the proper algorithm is critical for training data models. It is important to outline what is required from the prediction before using AI-based forecasting technologies. Furthermore, having a huge amount of data often produces better results because machine learning is all about teaching the machine to acquire insights using the data available.
Conclusion
In conclusion, AI/ML-enabled demand planning solutions can be a good option to solve these problems as they not only help businesses to achieve accurate forecasts but also help them to find hidden business values by leveraging the high amount of data they have. This article provides a clear understanding of the common errors and the parameters to be considered when forecasting. Therefore, it is advisable to use artificial intelligence (AI) based forecasting tools such as Thousense Lite, which helps in forecasting the best results and maximizing profits.