AI in Retail Demand Forecasting: Moving from Reactive to Proactive
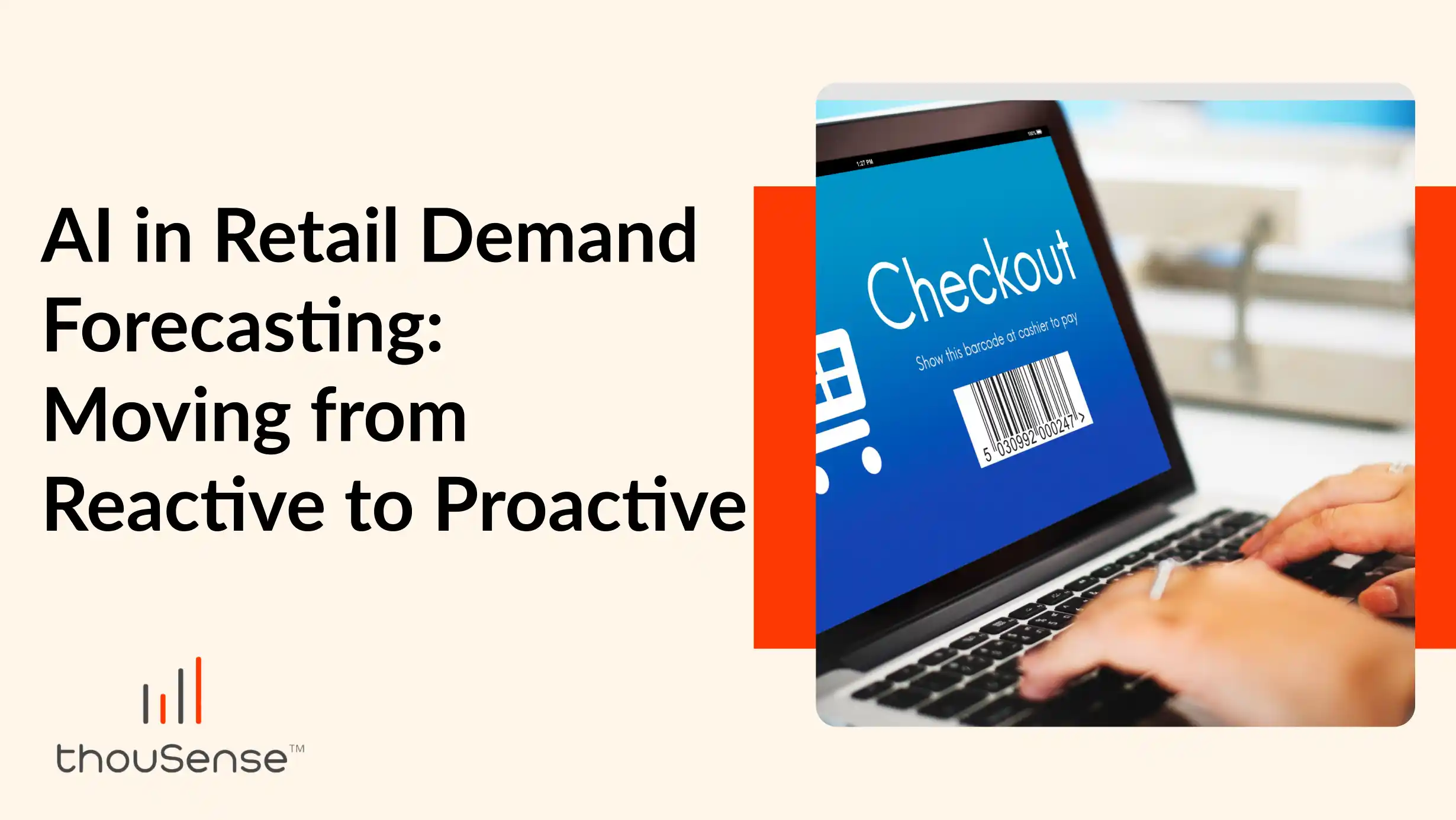
Introduction
In the dynamic world of retail, the art of predicting what, when, and how much customers will buy is crucial. Traditional demand forecasting methods, though reliable, often lag behind the fast-paced market trends. Enter AI in demand forecasting, a revolutionary approach that's reshaping the retail landscape. This blog explores how AI is transforming demand forecasting from a reactive process into a proactive strategy, ensuring retailers stay ahead of the curve.
The Essence of AI in Retail Demand Forecasting
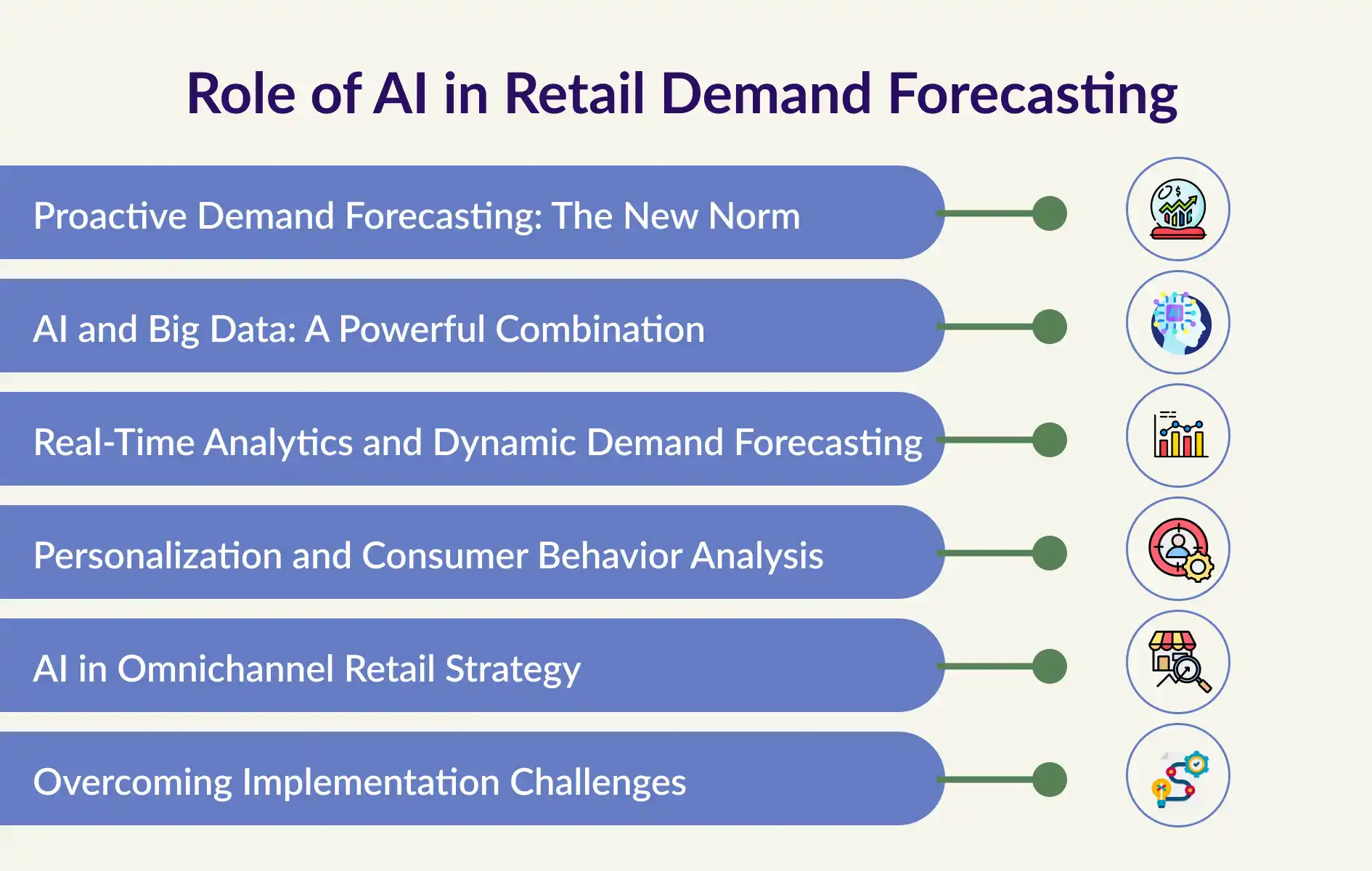
AI in demand forecasting represents a significant leap from traditional methods. AI uses machine learning and neural networks to analyze higher volumes of data and uncover hidden patterns. This advanced analysis translates into more accurate and efficient demand forecasting for retail, a critical advantage in today's competitive market. AI doesn't just predict; it learns and adapts, continually refining its forecasts. As per a report by McKinsey, organizations that have adopted AI for demand forecasting can reduce errors by 20-50%.
From Reactive to Proactive Shifting Gears in Retail Demand Forecasting
The transition from reactive to proactive demand forecasting in the retail sector marks a significant paradigm shift, fueled by Artificial Intelligence (AI) integration. This transformation transcends traditional methods, empowering retailers to anticipate market changes and customer needs with unprecedented accuracy and speed.
Proactive Demand Forecasting: The New Norm
In the reactive model, retailers traditionally responded to market changes and consumer behavior after they occurred. This approach often led to inventory mismanagement, either through overstocking or stockouts, and missed opportunities. The proactive model, powered by AI, changes this narrative. Retailers can now predict market trends and customer preferences in advance, allowing for strategic decision-making that aligns inventory and marketing efforts with anticipated demand.
AI and Big Data: A Powerful Combination
Big data is the fuel that powers AI's engine in demand forecasting. By integrating diverse data sources, including social media trends and economic indicators, AI provides a holistic view of market dynamics. This comprehensive approach enables retailers to make informed decisions, aligning their strategies with real-world scenarios. Big data isn't just about quantity; it's about harnessing quality insights for better demand forecasting.
Real-Time Analytics and Dynamic Demand Forecasting
The retail market is ever-changing. In such a scenario, the AI's ability to process real-time data is a game-changer. Dynamic forecasting allows retailers to adjust their strategies on the fly, responding to immediate market shifts. This agility is crucial for inventory management and supply chain optimization, reducing the risks of overstocking or stockouts. For instance, Amazon's dynamic pricing algorithm adjusts prices every 10 minutes based on real-time demand, significantly optimizing their inventory levels.
Personalization and Consumer Behavior Analysis
In a survey by Forbes, it was found that 81% of customers are more prone to make a purchase when brands offer personalized experiences. AI goes beyond mere numbers; it delves into the realm of consumer behavior. By analyzing purchasing patterns and preferences, AI helps retailers tailor their offerings, ensuring that the right products reach the right customers. High-level personalization improves the customer experience, promoting loyalty, and repeat business.
AI in Omnichannel Retail Strategy
In today's retail, the lines between online and offline shopping are blurring. AI plays a pivotal role in balancing demand forecasting across multiple channels. This omnichannel approach ensures a seamless customer experience, whether shopping online or in-store. Retailers can manage inventory more effectively, meeting customer demands wherever they choose to shop.
Overcoming Implementation Challenges
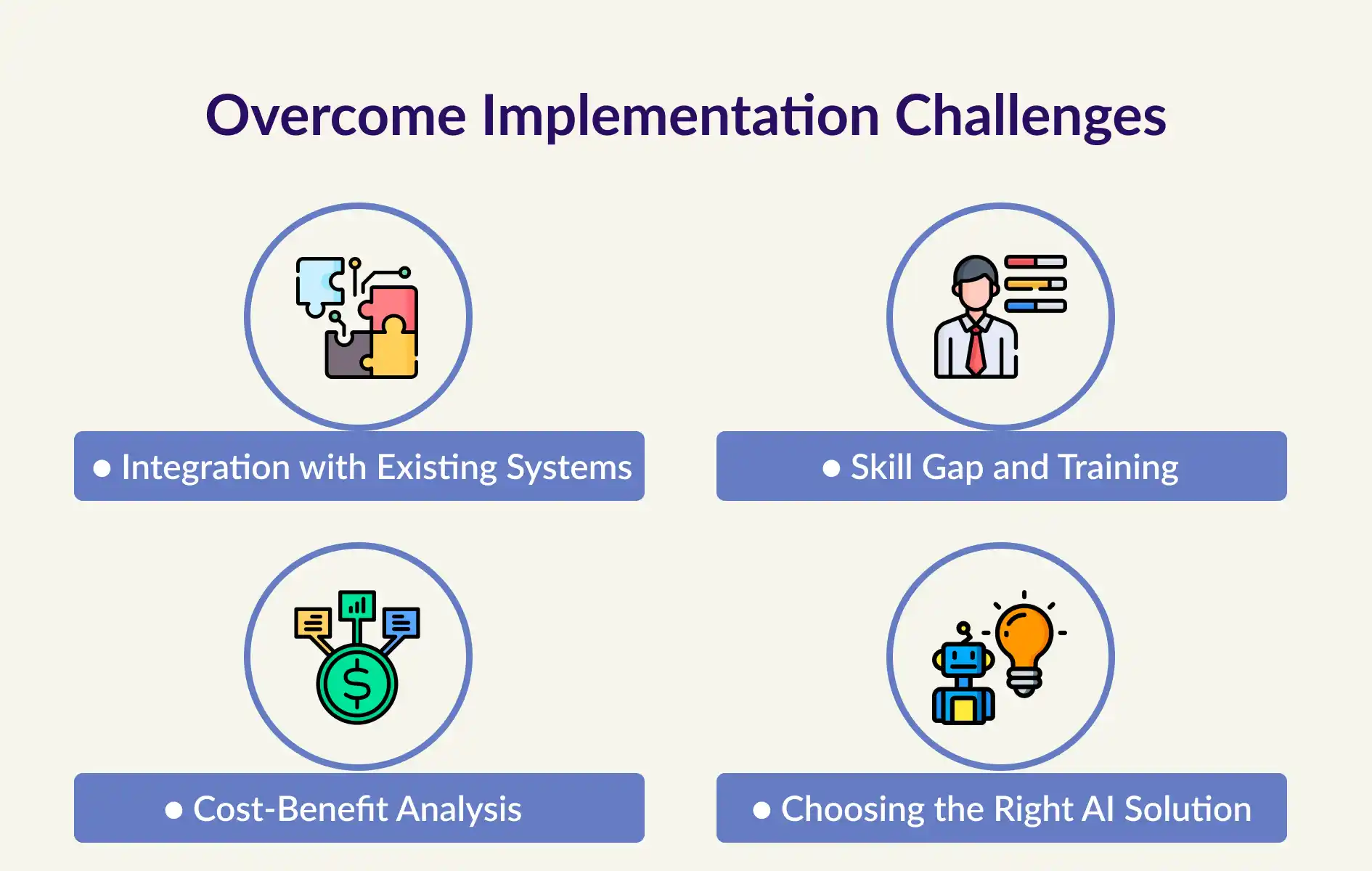
Adopting AI in demand forecasting is not without its challenges. Retailers must navigate integration issues and ensure their teams are equipped to manage AI-driven systems. However, the benefits far outweigh the hurdles. With the right strategy and support, implementing AI can lead to significant improvements in demand forecasting accuracy and efficiency. The following are some ways implementation challenges can be overcome:
● Integration with Existing Systems: Discuss the technical challenges of integrating AI solutions with legacy systems. Emphasize the need for a scalable and flexible infrastructure.
● Skill Gap and Training: Highlight the importance of upskilling existing staff or hiring new talent with expertise in AI and machine learning. Training sessions and workshops can be instrumental in smoothing the transition.
● Cost-Benefit Analysis: Offer insights into the initial investment costs versus long-term benefits. Retails must establish a minimum ROI that they can expect from implementing AI in demand forecasting.
● Choosing the Right AI Solution: Guide on selecting appropriate AI tools and vendors, considering factors like scalability, support, and customization options.
thouSense - Pioneering AI in Retail Demand Forecasting
In this unpredictable retail sector, thouSense is emerging as a frontrunner in advancing AI-driven demand forecasting. Leveraging cutting-edge AI technologies, thouSense's platform is redefining how retailers approach the challenge of predicting market demands.
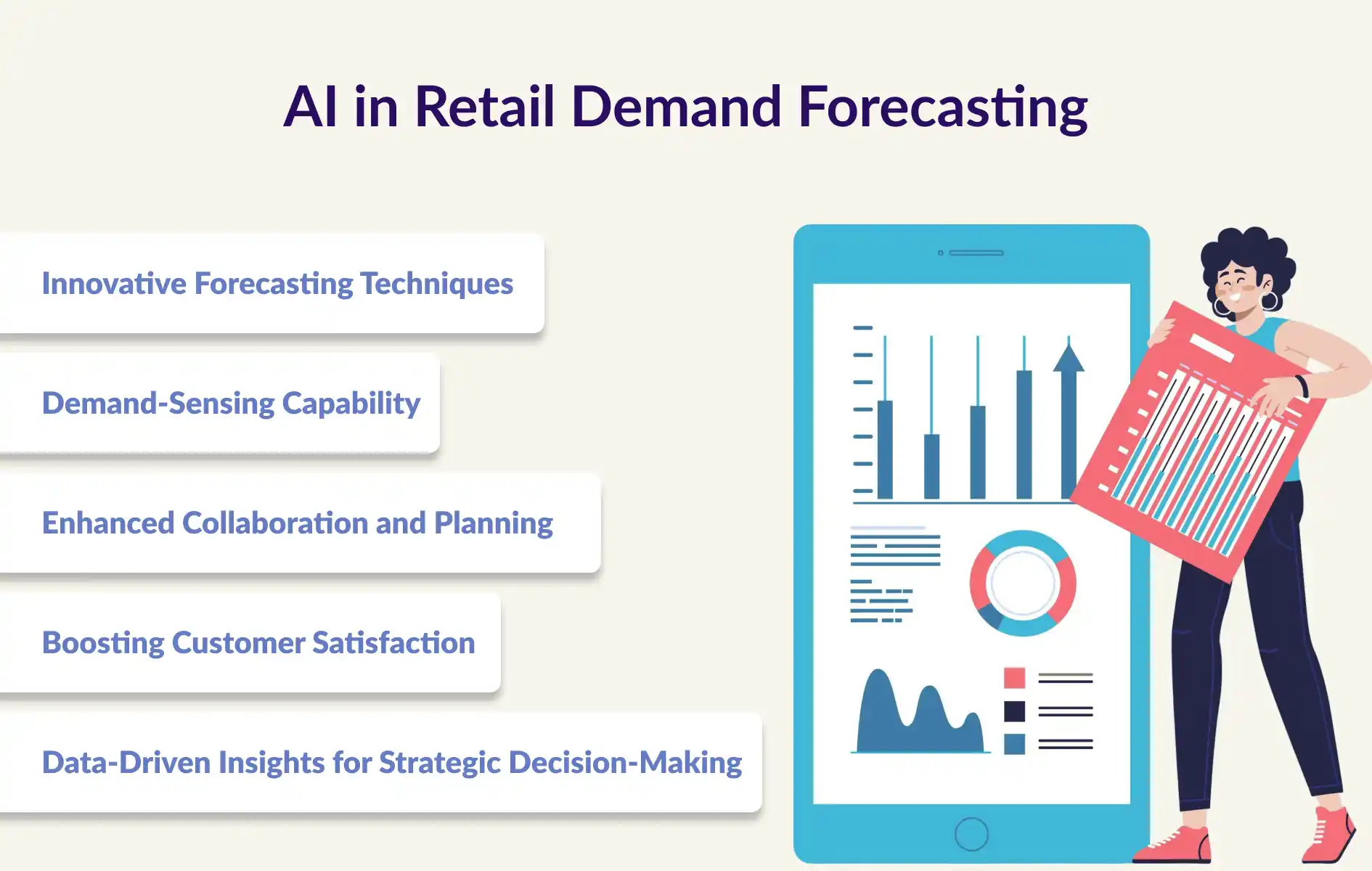
Innovative Forecasting Techniques
thouSense employs advanced machine learning and deep learning algorithms, which enable the platform to generate precise short-to-long-term forecasts. These algorithms consider a wide array of factors, including seasonal trends, promotional impacts, and external market variables. This sophisticated approach ensures accurate forecasts. it also makes such forecasts highly adaptive to changing market conditions.
Demand-Sensing Capability
A key differentiator for thouSense is its demand-sensing feature, which allows retailers to identify shifts in demand patterns much earlier than traditional methods. By integrating data from various sources such as social media, weather reports, and economic indicators, thouSense can pinpoint emerging trends, enabling retailers to take preemptive actions.
Enhanced Collaboration and Planning
thouSense promotes cross-functional collaboration within organizations. Its unified platform bridges the gap between different departments, fostering seamless integration and communication. This coordination is crucial for effective demand planning and smoother execution in Sales and Operations Planning (S&OP).
Impact on Retailers: With thouSense, retailers can achieve enhanced inventory management, leading to optimal stock levels and minimized risk of stockouts or overstocking. This precision in inventory management directly translates to improved cash flow and reduced inventory costs.
Boosting Customer Satisfaction
At the heart of thouSense's capabilities is a focus on customer satisfaction. By ensuring that products are readily available and market needs are proactively addressed, thouSense aids retailers in enhancing customer loyalty and satisfaction.
Data-Driven Insights for Strategic Decision-Making
Beyond forecasting, thouSense provides a comprehensive dashboard of Key Performance Indicators (KPIs). This allows retailers to track performance, identify trends, and make informed, data-driven decisions. Therefore, it gives them a competitive edge in the unpredictable retail market.
The Future of AI in Retail Demand Forecasting
The future of retail demand forecasting is undeniably intertwined with AI. As technology evolves, we can expect even more sophisticated AI applications, further enhancing the accuracy and effectiveness of demand forecasting. Retailers who embrace AI today are setting themselves up for success in the ever-evolving retail landscape.
Emerging AI technologies like deep learning and predictive analytics offer intrigue. They could further refine demand forecasting by providing a much more rooted insight into the markets and retail processes. Moreover, AI can aid in creating sustainable supply chain practices. In this way, AI can even help in waste reduction and further betterment in the efficiency of the supply chain.
Finally, AI will offer hyper-personalized shopping experiences. It will use data not just to forecast demand but to shape product development and marketing strategies.
Conclusion
The implementation of AI into retail demand forecasting goes beyond being just a trendy technology. It is an evolution in how companies approach market dynamics. The change from a reactive to a proactive stance in forecasting is not just about how to become more competitive. Still, it involves changing strategies completely based on quickly developing consumer behaviors and market trends. Demand forecasting plays an essential role in this transformation, where AI provides unparalleled levels of precision, experience, and adaptability.
Retailers implementing AI can predict demand with more accuracy and respond quickly to market changes, resulting in efficient inventory management and improved customer satisfaction. AI helps retailers offer a more personalized experience than operational efficiency as it leads to a deeper understanding of consumer preferences. This is not the only benefit of this change; it nurtures loyalty and develops a more invigorating, fulfilling relationship between the retailer and the customer.
As for the future, innovation in the retail demand forecasting landscape of AI is also on its way. The increasing adoption of emerging technologies, including deep learning and predictive analytics is set to present even more profound knowledge of consumer behavior patterns as well as market trends. These innovations are not simply evolutionary; they can reset retail effectiveness, sustainability, and customer focus.
FAQs
1. What is retail demand forecasting?
Retail demand forecasting is the process of predicting customer demand to optimize inventory, sales, and business planning in retail.
2. How to do retail forecasting?
Retail demand forecasting involves using historical sales data, analyzing trends, considering seasonality and external factors, and leveraging AI tools.
3. What are the components of retail forecasting?
The key components of retail demand forecasting are historical data, seasonality, trends, external factors, and technology-driven tools.