Seasonality Challenges in Demand Forecasting
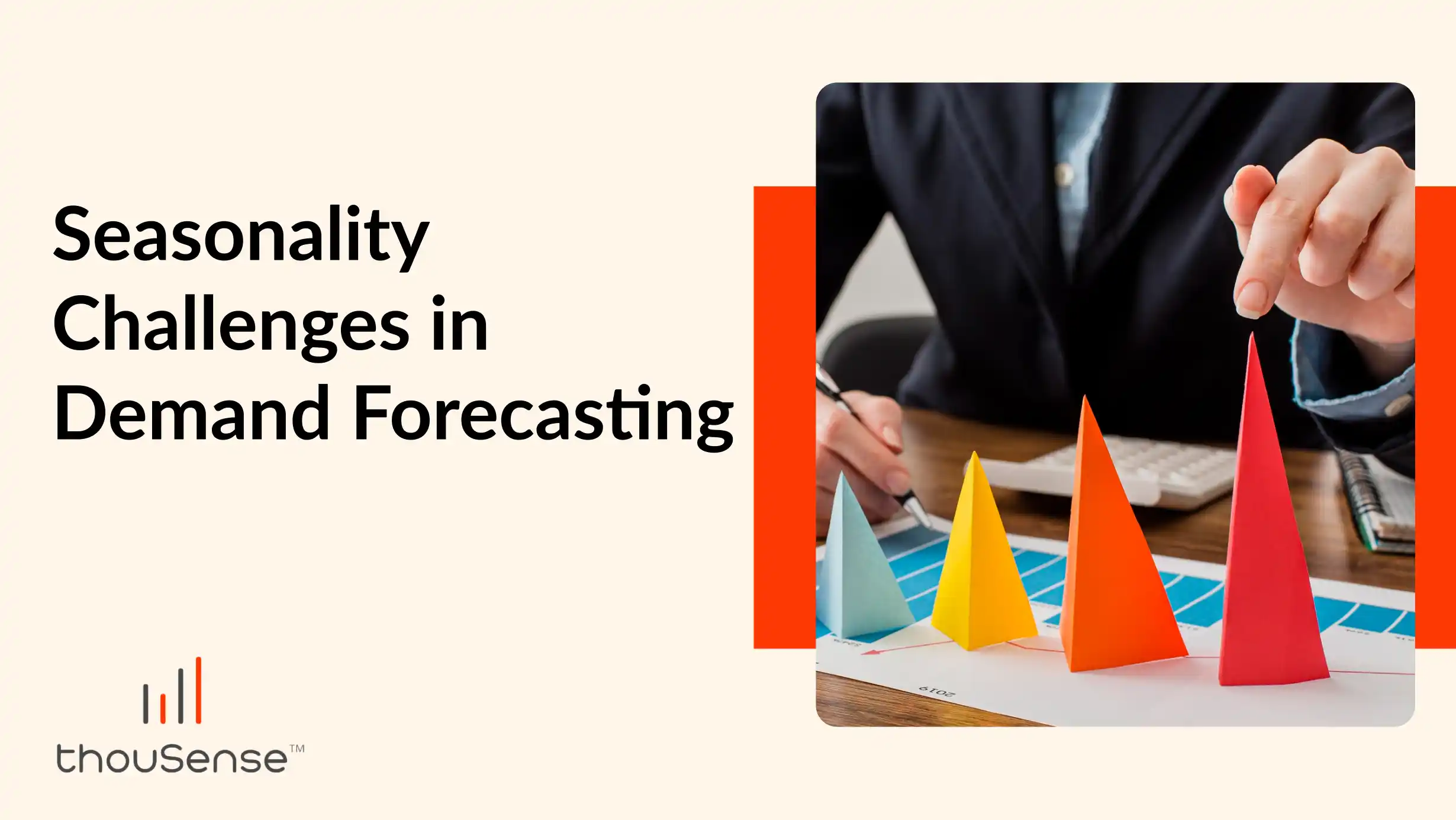
In the world of business, forecasting demand plays a crucial role. It helps companies prepare for what customers might need, allowing them to manage their resources effectively. Yet, predicting demand becomes a bit tricky when it comes to seasonal patterns. Seasonality means the expected fluctuations in demand that happen during certain times of the year, like holidays, weather shifts, or cultural festivities.
Knowing these seasonal patterns is vital for all sorts of businesses, whether they're in retail, online shopping, making products, or farming. When companies can accurately guess how demand changes with the seasons, they can plan their stock, production, and resources better. This preparation helps them avoid shortages or excess stock, keeping their operations running smoothly.
Identifying Seasonal Trends
Identifying seasonal trends is a vital step when dealing with seasonal challenges. It means studying past data to understand how sales or demand changed during different times of the year. The aim is to find patterns that repeat and help us guess future demand. To do this, we often use statistical methods like time-series analysis and exponential smoothing.
● Time-Series Analysis
Time-series analysis looks at how things changed over time by dividing data into periods like months or quarters. This helps us see how demand fluctuates during different times.
Overview of Time Series Analysis:
1. Time series analysis studies historical data to identify patterns and trends over time.
2. It focuses on analyzing data points collected at regular intervals, such as daily, monthly, or yearly.
3. The primary goal is to uncover underlying patterns, including seasonal fluctuations, trends, and irregularities.
4. Commonly used methods in time series analysis include moving averages, decomposition, and autocorrelation.
5. Seasonal trends can be detected by examining how data points vary across different seasons or time periods.
6. Time series analysis helps businesses forecast future demand or sales based on historical patterns, aiding in decision-making and planning.
● Exponential Smoothing
Exponential smoothing smooths out the bumps in data to show the main trends. It gives more importance to recent data to highlight current patterns. This helps us see the main seasonal trends.
Overview of Exponential Smoothing:
1. Exponential smoothing is a statistical technique for analyzing time series data.
2. It smoothens out data irregularities to reveal hidden trends.
3. It gives more weight to recent data, making it more important.
4. Exponential smoothing helps to find and predict trends by lessening random changes.
5. Seasonal trends can be seen by using exponential smoothing on seasonal data.
6. This technique is really helpful for guessing future demand or sales because it shows a smoothed version of past patterns.
7. Exponential smoothing helps businesses decide on inventory, production, and resources.
Both time-series analysis and exponential smoothing are truly helpful. They give us insights into seasonal trends, so we can make smart decisions about things like how much stock to keep or when to produce more. Knowing these patterns helps companies avoid running out of stock or having too much left over.
Challenges in Demand Forecasting Due to Seasonality
Forecasting demand is a big challenge for businesses, especially when it comes to seasonal changes. In this section, we'll explore the difficulties businesses face in predicting how much people will buy during different times of the year. From understanding customer behavior to managing stock and dealing with competition, we'll break down the complexities of anticipating demand in the ever-changing world of season
1. Navigating Customer Behavior
Understanding how customers change preferences based on seasons poses a big challenge. For instance, in summer, they might prefer outdoor activities, while in winter, they may opt for indoor pastimes. This makes it hard for businesses to predict how much of their product people will want, leading to either too much or too little stock.
2. Dealing with Unstable Data
Seasonal demand creates data that's hard to predict. Traditional methods struggle with these fluctuations. Additionally, unexpected events like bad weather or economic changes make it even harder to guess what people will buy.
3. Balancing Stock Management
Businesses need to get the right amount of stock for seasonal demand. They want enough to meet higher demand during peak times but not so much that they're left with leftovers when the season ends. Getting this balance right is tough and can lead to missed sales or wasted stock.
4. Challenges in Forecasting
Standard ways of predicting demand might not handle seasonal changes well. For example, simple methods like looking at averages or using straight lines might miss the fluctuations of seasonal demand. Businesses need more complex methods that account for these fluctuations.
5. Strains on the Supply Chain
Seasonal demand can strain the supply chain. Suppliers might struggle to make enough when demand is high, causing delays or shortages. Transport and delivery networks might also get stretched thin, leading to longer wait times and higher costs.
Strategies for Addressing Seasonality Challenges
To handle changes in demand throughout the year, businesses can use different strategies. One helpful approach is using advanced forecasting models like time-series analysis and exponential smoothing. These models look at past data to see how demand has changed over time. By understanding these patterns, businesses can make better guesses about future demand.
It's also useful to bring in industry experts who know a lot about seasonal trends, what customers like, and how the market works. This expertise can improve the accuracy of forecasting models.
Another good idea is to work closely with suppliers, distributors, and retailers. By involving them in the forecasting process, businesses can get more insights and data from different parts of the supply chain. This helps to understand what drives demand and improves forecasting accuracy.
Collaboration also leads to better coordination across the supply chain, which helps with inventory management and operations. By sharing resources and knowledge, businesses can anticipate changes in demand and adjust their plans accordingly.
Investing in technology and data analysis tools can further improve forecasting accuracy. These tools can quickly analyze large amounts of data to find patterns and trends. By using these insights, businesses can make smarter decisions and improve their forecasting processes.
Using advanced forecasting techniques, industry expertise, collaboration, and technology can help businesses manage seasonal changes better, leading to improved efficiency and performance.
Conclusion: Mastering Seasonal Demand Forecasting
In conclusion, dealing with seasonal changes in demand forecasting needs a good grasp of seasonal trends, using data, and having smart plans. By recognizing seasonal patterns, tackling forecasting problems, and using advanced analysis, businesses can improve their forecasting and predict changes in demand better.
Understanding seasonal demand forecasting isn't just about managing inventory and resources well. It's also about meeting customer needs and making more money. With the right tools, methods, and knowledge, businesses can handle the challenges of seasonal demand forecasting and succeed in today's fast-changing market.
FAQs
1) Why is understanding seasonal demand important for businesses?
Seasonal demand affects inventory management and resource allocation, crucial for meeting customer needs and maximizing profits.
2) How do external events impact seasonal demand forecasting?
Economic downturns, natural disasters, and pandemics can disrupt demand patterns, challenging businesses to adapt quickly.
3) What techniques are used to identify seasonal trends in demand?
Methods like time-series analysis and exponential smoothing analyze historical data to reveal recurring patterns and aid in forecasting.
4) How can businesses overcome challenges in seasonal demand forecasting?
By investing in advanced forecasting models, leveraging industry expertise, collaborating across the supply chain, and embracing technology.
5) What are the implications of inaccurate seasonal demand forecasting?
Inaccurate forecasts can lead to stock shortages, excess inventory, missed sales opportunities, and ultimately, reduced profitability for businesses.