5 Reasons Why You Should Use Machine Learning for Forecasting Demand
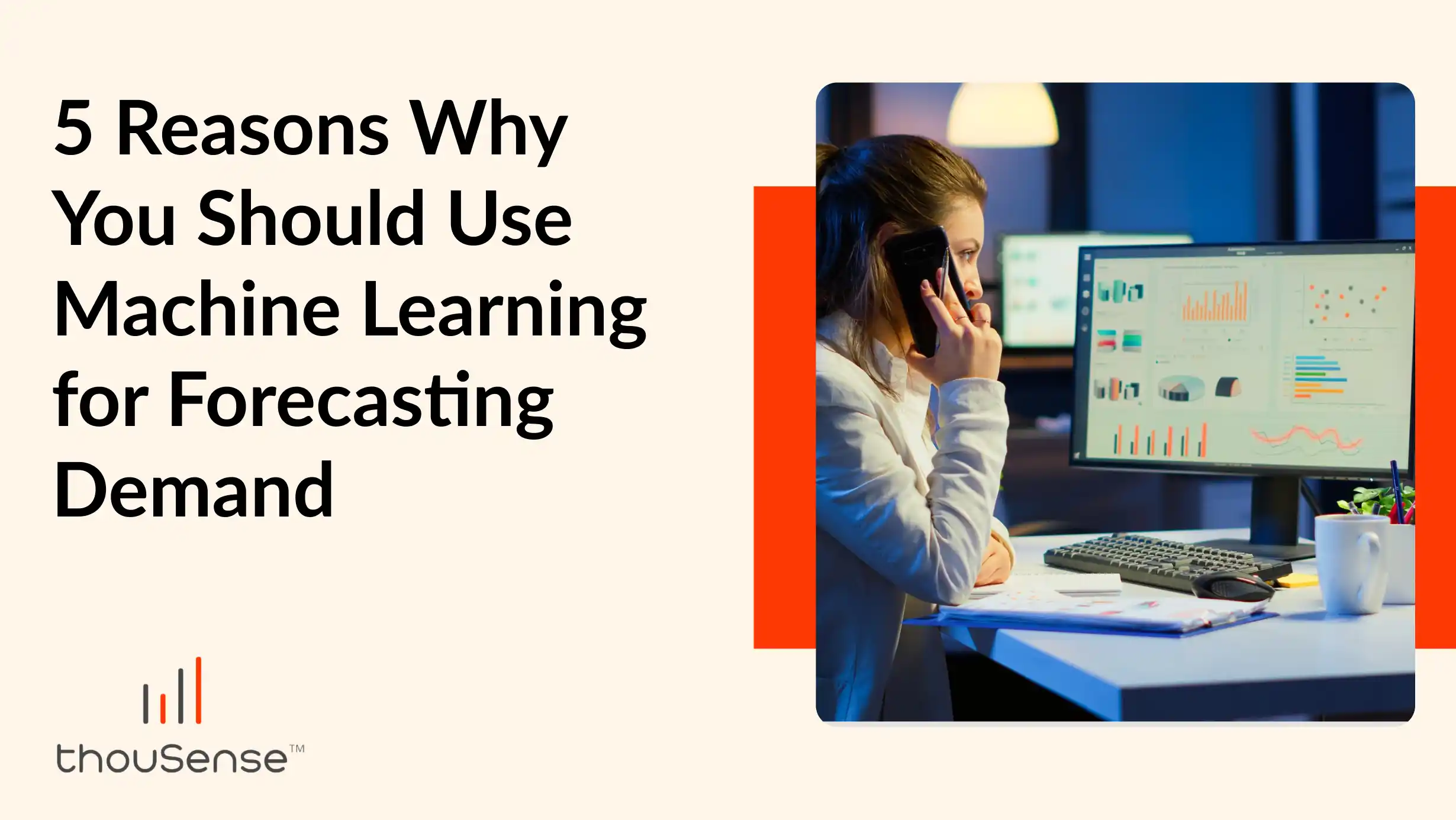
Demand Volatility has been one of the biggest pain points for businesses. Machine learning has proven to have helped demand planners increase their forecast accuracy beyond what was achievable via a mixture of traditional statistical methods and human intuition.
There’s also a thumb rule that suggests that one can reduce your planned inventory by 2.5% with a 1% improvement in the inventory forecast. Utilizing machine learning for demand forecasting clearly has the potential to improve service levels by preventing stockouts and reduce the overall inventory being carried by the company as the forecast accuracy increases.
While the above in itself is a compelling reason for companies to try out machine learning to generate their demand forecasts, below are our top 5 reasons why you should use Machine learning for demand forecasting:
1. Quick to get a baseline demand forecast
Utilizing machine learning for demand forecasting has fast become a way for demand planners to quickly get to a baseline forecast and utilize it to further improve their forecasting accuracy in the demand planning process.
To achieve this, all you require is your historical demand data and let the ML models work their magic to get you a forecast in minutes/hours, depending on the volume of your data.
2. Ability to use exogenous variables (weather/price/macroeconomic data) to predict demand
For smaller companies (MSMEs) who do not yet have a demand planning team or a process in place beyond the rolling moving average methodology for forecasting future demand, machine learning tools for demand forecasting can help by utilizing data beyond just the internal sales/demand data. Depending on the industry, external factors like macroeconomic data as well as weather data play a key role in determining the forecast in both the short and the long term.
3. Forecasting for highly volatile SKUs
While traditional statistical models can predict the demand of SKUs with stable demand, they do not perform well for highly volatile SKUs. Identifying complex patterns, trends, and relationships between multiple variables (internal or external) that lead to better forecast accuracy are only achieved via utilizing complex Machine Learning and Deep Learning Models.
4. Eliminates Human Judgment and bias in the forecast
A lot of demand forecasts developed today are prone to human judgment and bias of the person generating the forecast. Utilizing Machine Learning tools for demand forecasting eliminates the bias from the forecast. Also, as more data gets fed into the machine learning model, the more it learns and tends to give better results over a period without any inherent bias.
5. Ability to rapidly adapt to change and supply chain disruptions
ML tools, unlike demand planners, can generate forecasts repeatedly, while adapting to the most recent data of actuals as soon as they are fed to the tool. Since regenerating forecasts based on the most recent actuals is swift and always available via the click of a button, demand planning teams can utilize the forecast that has the context of the most recent data of actuals. This can then be leveraged to adjust the demand plans due to any disruptions that may have happened.
Looking for a Machine learning tool for demand forecasting?
Contact us to get started